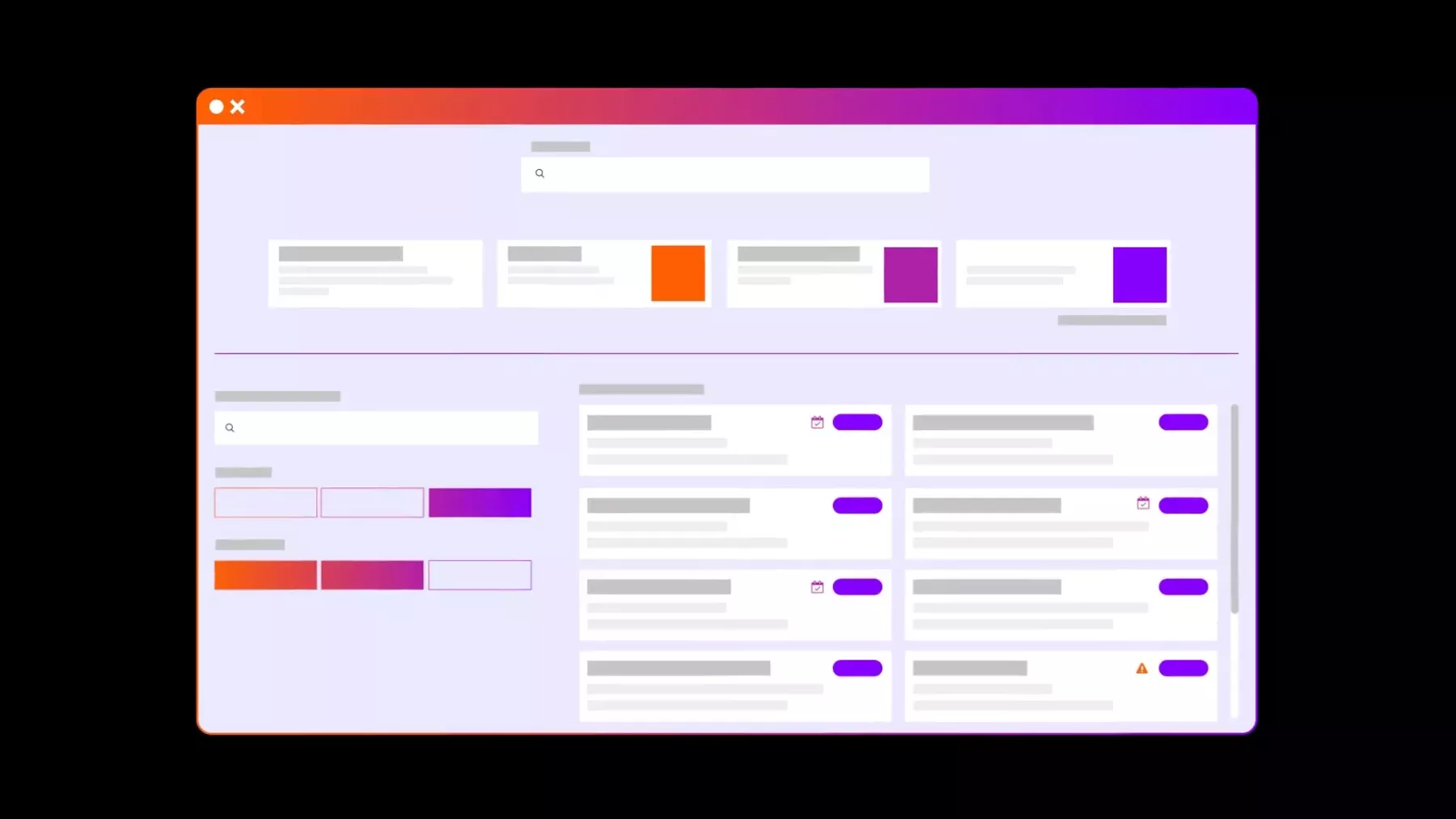
The data lakehouse built for healthcare
Arcadia is a leading cloud-based healthcare data platform purpose-built for analytics. Deliver better outcomes, reduce medical expenses, and spark innovation faster than ever with a data architecture primed for healthcare’s future.
Bad data can lead to serious consequences in healthcare
Delivering insights to the right people at the right time can mean life or death. Because of this, data is the key to success in healthcare, unlocking productivity for care teams and better lives for patients. So why do so many healthcare organizations struggle to utilize their full data asset?
The right data platform unlocks insights across the care continuum
Transformative healthcare leaders know that data is as diverse as the people it serves. Without the right architecture, good data gets trapped, locked in place, without purpose. That’s why they leverage Arcadia’s next-gen data platform to accelerate outcomes, amplify impact, and deliver rapid insights to thousands of users across their organizations.
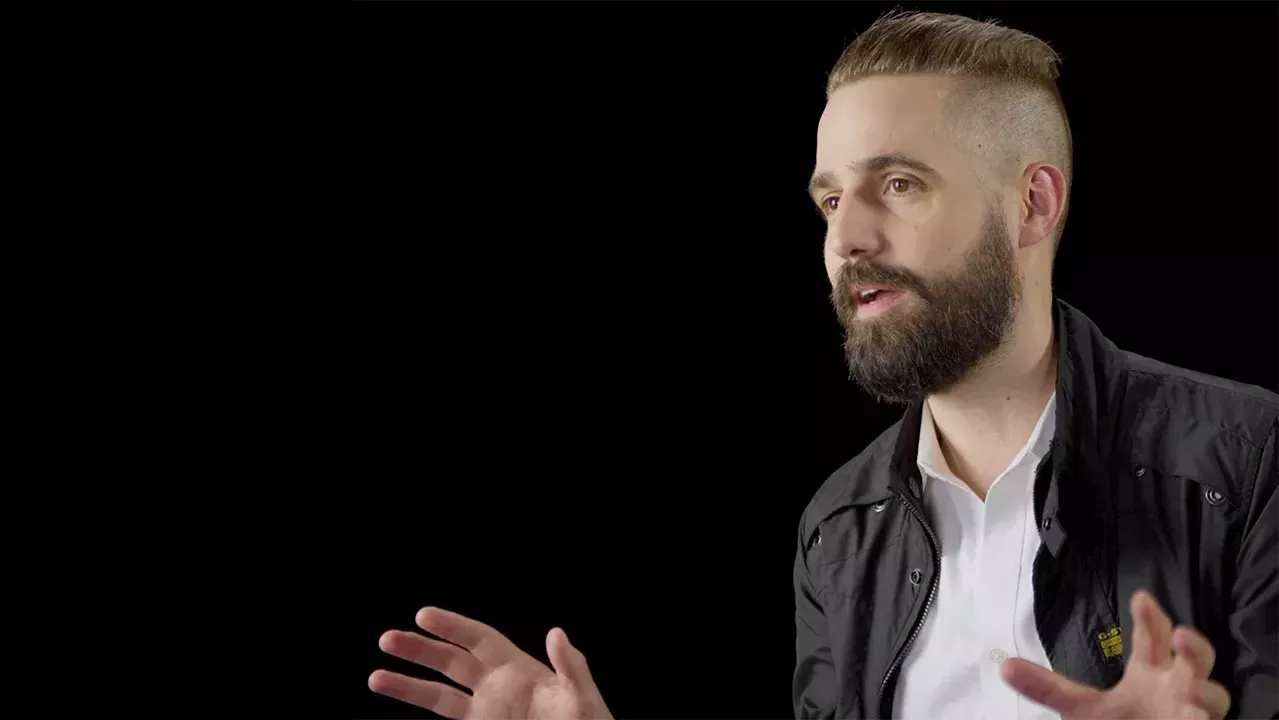
A data platform built for the complexity of healthcare
Unify disparate data sources, get a more comprehensive view of patients, and power AI models, algorithms, reports, and dashboards to make better informed decisions — all on one platform. Listen in as Arcadia CPTO Nick Stepro explains how the data lakehouse empowers transformative outcomes for modern healthcare organizations.
Get a demo of Arcadia’s data platform
Get in touch to see how this data platform will transform your healthcare organization.
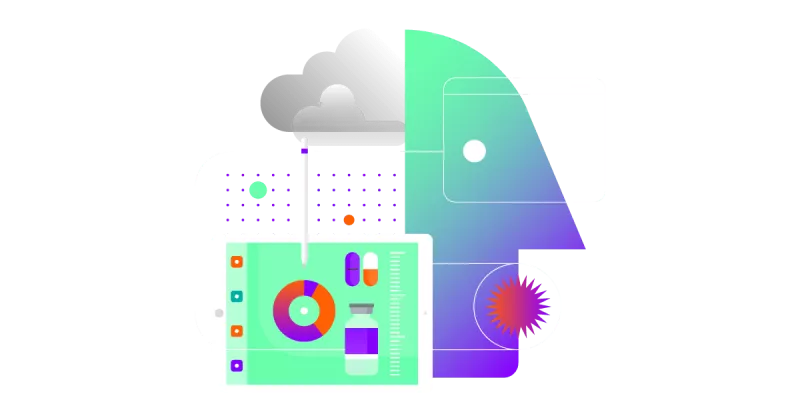
Analytics for forward-thinking healthcare organizations
A single hospital can produce upwards of 50 petabytes of data annually. As much as 95% of that goes unused. Leading healthcare organizations aggregate their data in Arcadia to keep patients healthy, determine treatments, and manage chronic disease.
Arcadia has empowered customers to achieve:
More closed risk per patient
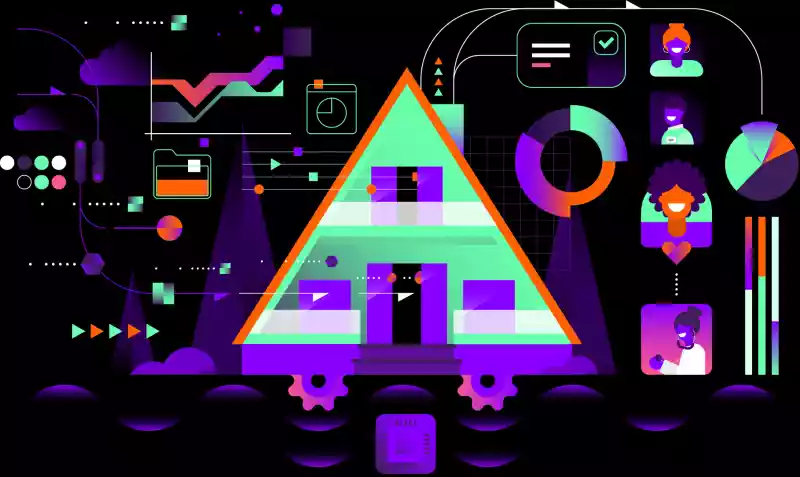
A foundation for success in the business of care
Success starts with an enterprise healthcare data asset your team can trust. Arcadia curates data from clinical EHRs, claims, social determinants of health (SDoH), pharmacy records, ADTs, and other sources on a data lakehouse platform built for healthcare. This solid foundation enables lasting financial success in the dynamic world of healthcare and value-based care.
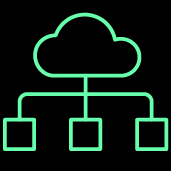
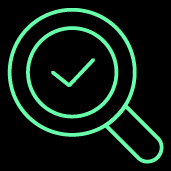
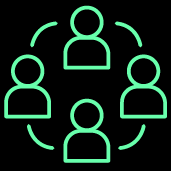
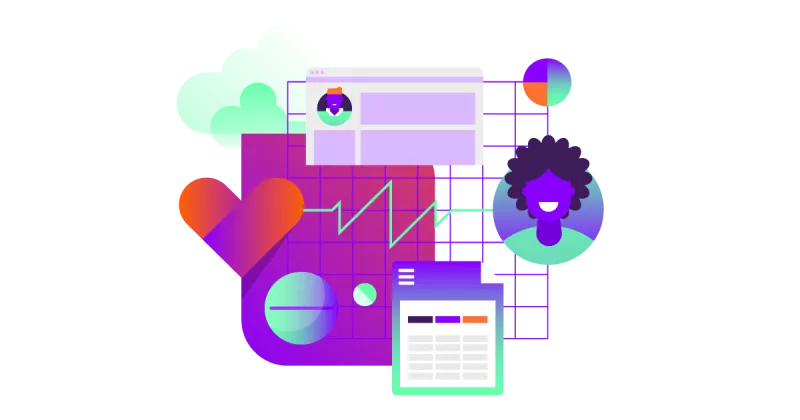
An intelligence platform built for the future of healthcare
Arcadia is a cloud-based, HIPAA-compliant data platform built to unlock insights from point of care to population health. Healthcare organizations use Arcadia to drive success under MSSP, NextGen, Medicaid, fee-for-service, and commercial value-based care contracts.
Arcadia Assess
Simplify provider-payer collaboration for risk and quality gap closure
Arcadia Bindery for Providers
From provider reporting to efficient healthcare data analysis
Arcadia Bindery for Payers
Health plan and payer reporting delivered through secure email and web-based apps
Arcadia Care Manager
Reduce healthcare risk and cost with data-driven care management
Arcadia Desktop
Find health insights at the point of care using your existing EHR workflows
Arcadia Engage
Automate patient engagement with AI-powered care management tools
Arcadia Engage Plus
Analytics meets patient engagement in our partnership with Artera
Arcadia Foundry
Healthcare data model built by analysts, for analysts
Arcadia HCC Risk Suspecting App
HCC risk suspecting software integrated with Epic for risk adjustment
Arcadia Patient Registry
Quickly build cohorts, stratify patients, and close more gaps
Arcadia Referrals
Data-driven physician referral management for healthcare organizations
Arcadia SDoH Package
Improve health outcomes by streamlining the way you identify populations most in need of care
Arcadia Vista
Business intelligence software built for healthcare organizations
Arcadia’s data platform is somehow the most valuable thing we have. This data asset that we built with Arcadia is the foundation for all our analytics and all the other work we do. We have found it to be scalable, stable, and flexible. We integrate not only very normalized clinical and claims data sets but also a lot of other data. And Arcadia can handle that and present that data. If every product that Arcadia sells were to go away completely tomorrow, we would still want to have this data asset. We spent years building it, and Arcadia does a very good job. I would dare anybody to go to another vendor and have them try to integrate the level of data that we have integrated with Arcadia. Their data platform always blows me away, and Arcadia deserves credit.
Get a demo of Arcadia’s data platform
Get in touch to see how this data platform will transform your healthcare organization.