A closer look at the evolution of diabetes
Our data visualization, The Evolution of Diabetes, traces patients over 12 years as they improve their health or develop complications — and demonstrates the power of predictive analytics for population health
The number of adults in the United States between the ages of 18 and 79 with newly diagnosed diabetes more than tripled from 493,000 in 1980 to over 1.4 million in 2014. Diabetes is an important condition to manage, as the burden of suffering is enormous and the downstream medical complications can be very severe.
Patients with diabetes can benefit from a range of treatments, such as medication, nutritional management, physical activity, glucose monitoring, and behavioral health support. Because these treatments require ongoing patient engagement, early and ongoing patient education is key to success. This means early identification of and outreach to at-risk patients is an important capability for a diabetes management program.
EHR and claims data can be used to identify at-risk patients. Arcadia’s Benchmark Database, a de-identified dataset of Claims and EHR records for 18 million lives, gives us the ability to analyze longitudinal data in order to predict whether a patient will develop diabetes.
What do diagnosis transitions mean?
In this data visualization by Arcadians Abhi Kumbara, Amy Werner-Allen, and Michael Simon, over a decade of EHR data for diabetic patients are normalized to visualize transitions between various states of severity and control of the condition in a Markov model.
- Rising red lines represent patients who are initially diagnosed with diabetes or whose diagnosis increases in complexity, as defined by lab and assessment data from the EHR.
- Swooping green lines show patients going in the opposite direction, moving into a position of better condition management.
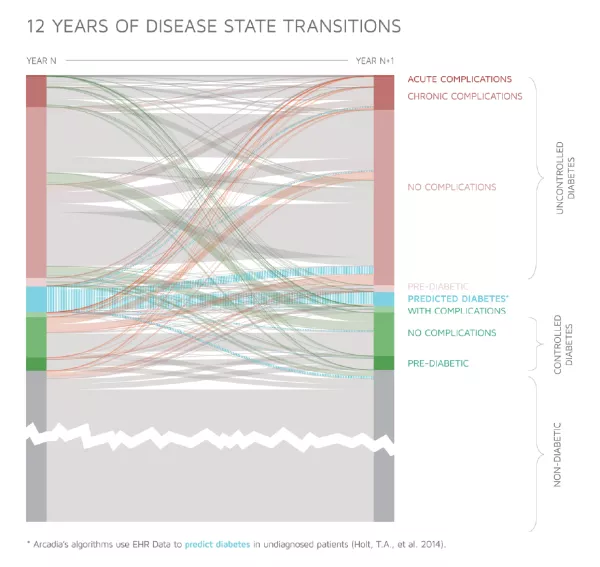
The reasons behind these transitions vary — among them, medication adherence, complicating conditions and unplanned (or well-managed) social, psychological or economic interactions.
A successful diabetes management program would transition as many patients as possible into a better state of condition management. Algorithms can help identify patients at different levels of condition severity — including patients who have not yet been diagnosed. This algorithm uses EHR data to identify undiagnosed patients who will become diagnosed diabetes patients.
Substantial number of the identified at-risk patients were diagnosed the following year
In this longitudinal study, Arcadia implemented a predictive algorithm that leverages a variety of lab result data to identify undiagnosed diabetics, a group that the CDC estimates represents over 25% of all diabetics nationally.
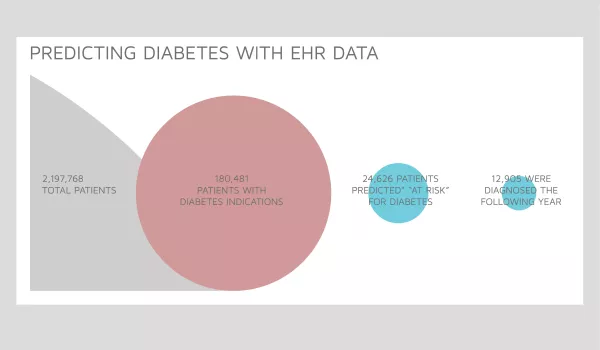
The algorithm identified a group of patients — over 13% of the size of the known diabetic population — at a high likelihood of being diagnosed with diabetes in subsequent years. Forty-five percent of those patients were diagnosed the following year. The vast majority were uncontrolled at the end of that year.
The incidence of new cases was 7.8 per 1,000 individuals in 2012. This rate can be used as a baseline to judge the effectiveness of our prediction. Using the algorithm and the Arcadia Benchmark Database, we were able to identify a population that was diagnosed with diabetes at a substantially higher rate.
Possibilities for the future
Predictive analytics can help improve the accuracy of diagnoses and support early identification of at-risk patients. Early identification and intervention can result in better outcomes for patients, contributing to successful population health management.
Arcadia Analytics gives providers and care managers the ability to analyze longitudinal data, which can help trigger early outreach and education, increasing disease control rates.
Arcadians Abhi Kumbara, Amy Werner-Allen, and Michael Simon, PhD created this Markov model data visualization in 2015, using the Arcadia Benchmark Database. Abhi and Amy are technical data analysts who help Arcadia Analytics customers extract rich, high-quality clinical information from their EHRs. Michael was Principal Data Scientist at Arcadia.