Key components of a healthcare data analytics platform
In the quest for healthcare data analytics, it’s essential to know the major functions a platform can provide, and how they enable your goals
Healthcare runs on data. Like a pointillist painting, these discrete bits of information form a whole, and when they’re arranged in a way that makes sense, they give healthcare organizations a full, contextualized view. So how can you form a massive amount of data points into a coherent picture?
The answer is a data platform. In a saturated market, there are numerous choices, but to choose the right solution, it’s critical to understand their key functions. Here, we look at what you can expect from a healthcare data platform, from storage to user interfaces. Read on to learn how you can discern what your organization needs most, and make an informed decision around which technology you’ll adopt.
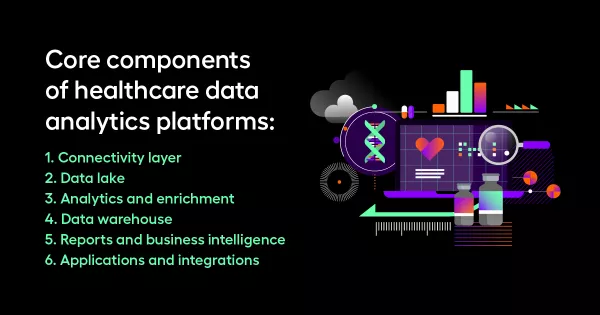
Core components of healthcare data analytics platforms
1. Connectivity layer
It all starts at the connectivity layer. Providers and administrators are inputting data every day, so at this preliminary step, the key question is: which data do you want to pull into a platform? And from there, another question follows: what are the business use cases you’re looking to support?
This dictates the data sources that someone would need to include, as well as fields needed to pull in. These uses could vary from regular reporting through ACO REACH to running a responsive, adaptable care management program (often, there are multiple within a single healthcare organization).
Then, it helps to address the volume of data that will be necessary to glean relevant insights. Not all data platforms can handle massive quantities of data, so the options will depend on that quantity and pace. Arcadia manages petabytes of data on behalf of some customers, and in practice, that sometimes looks like multiple days of processing to load a historical data set. As new information comes in, incremental updates are necessary to keep everything up to date.
“Each of these data sources is a snowflake,” Arcadia Sales Director Ryan Rubino says. “It’s a little bit different.” Arcadia actively maintains over 2600 EHR and claims data sources on behalf of customers, and that practice has led to efficiency and creativity. In this vein, consider a vendor or in-house team’s ability to adapt quickly, because that will be easier with a team who’s had to do so many times in the past.
It’s also worth noting that, in a landscape where data integration varies source by source, not all platforms are created equal. At the connectivity layer, it’s critical that a data platform supports multiple approaches for ingesting data. Payers might have a unique extraction approach for claims and eligibility sources, while EHRs vary vendor by vendor. In that vein, it’s important to be realistic and clear on what a platform offers (and what additional ETL will be required to get it off the ground).
2. Data lakehouse
A data lakehouse represents an innovative approach in database architecture, merging the concepts of a data lake and a data warehouse. This fusion offers a comprehensive solution for data management. A data lake is akin to a vast reservoir, storing copious amounts of data in diverse forms, ranging from raw to structured. It's designed to handle the sheer volume and variety of data, making it a flexible choice for storing large datasets. On the other hand, a data warehouse is more structured and organized, primarily focused on storing data that has been processed and formatted for specific analytical purposes.
By merging these two methodologies, a data lakehouse offers a unique blend of benefits. It provides the extensive storage capacity and flexibility of a data lake, allowing for the accommodation of vast and diverse data sets. Simultaneously, it integrates the structured, query-optimized environment of a data warehouse. This combination enables users to manage and analyze large volumes of data more effectively.
In a data lakehouse, the data retains its native format until needed. It supports various data types, including structured, semi-structured, and unstructured data, making it versatile for different kinds of data analytics and business intelligence operations. Moreover, a data lakehouse often incorporates advanced features like machine learning, generative AI, real-time analytics, and data governance tools, further enhancing its capabilities beyond traditional data storage and processing systems.
This architecture is particularly beneficial in scenarios where organizations, like health systems, need to extract actionable insights from large, complex, and varied data sets while maintaining high performance and scalability. The lakehouse model thus represents a significant evolution in the field of data management, catering to the growing demand for agile, efficient, and comprehensive data analysis solutions in the digital age.
3. Analytics and enrichment
Once a heap of data is centrally stored in a data lake, then it’s time to dig into its meaning. Enrichment of this data, for a specific purpose aligned with an organization’s goals, is what will drive business value and performance. Enrichment includes quality measurement and calculations, risk suspecting, analytics for cost and utilization, and predictive analytics. These enriched data insights are the foundation for both understanding performance and identifying those opportunities to drive action and improvement. The interventions this enriched data prompts will depend on what a healthcare organization is trying to accomplish, whether that’s identifying and mitigating an SDoH concern or exceeding the terms of a risk-based contract. What matters most is that the data has been vetted and polished, so that when it’s accessed, it’s trustworthy and centrally stored for collaboration and insights.
Using multiple vendors means those insights might not be so central. In fact, it opens up the possibility for more failure points, as discrete functions of the data retrieval and storage process get outsourced to different entities. By contrast, a single vendor typically offers an integrated solution with components that work seamlessly together. It’s worth noting this when considering the key roles a healthcare data platform will play at your organization.
4. Data warehouse
Even after you’ve taken a preliminary look at what story the data tells, it needs to be cleaned up and made available for use by data analysts and other key users.
Warehouse is an apt term: think of storing millions of small objects, but needing to access or locate each one with ease. Once again, let the use cases that are top priority for your organization guide this process. Do you need to tease out lab results rapidly and often? Do you need to quickly segment populations to gather demographic data? A library makes another helpful comparison — you wouldn’t want a textbook on physics shelved right by the front door if, typically, you’re dashing inside to grab The Hobbit.
Looking for a data warehouse and winding up with a data lake is a major potential pitfall. Imagine, for example, that a group is hoping to target patients with symptoms of prediabetes for a nutritional intervention — it would be frustrating to find that a data warehouse served up every patient with a high A1C (including a huge group of Type 1 and 2 diabetics).
Having clarity from the beginning about which information you’ll regularly need can help you vet what’s on the market and discern the offerings that are a good (or bad) match.
5. Reports and business intelligence
The prediabetes vs. diabetic patient example also speaks to a healthcare data platform’s reports and business intelligence capabilities. A great solution will properly segment and stratify critical data, so users can surface what they need without the additional burden of a bunch of irrelevant information.
Often, analytical queries began as sweeping questions, and then an organization will discover a regular cadence or report that needs to run frequently. You might, for example, want to keep tabs on a high-risk set of hypertensive individuals, or monitor changes in scheduled visits for a group that’s housing insecure. In the search for a data analytics solution, your milage may vary.
Identifying these needs before signing a deal will help you veto the vendors who can’t deliver what you need, and critically, it will give you a realistic sense of time-to-value. An out-of-the-box solution lets you focus immediately on business questions or goals, whereas it often takes six to 12 months to build out a fully-functioning dashboard.
Trust in that platform partner’s expertise becomes especially important when you realize that modifying reports and data streams can disrupt data quality up- and downstream. If you work in value-based care, for example, finding a vendor with expertise in that specific area will matter, whereas an organization that’s strictly fee-for-service would require different capabilities. Essentially, if an organization discovers they need a certain type of information added to the data warehouse retroactively, it can’t just be patched last-minute. The data has to flow in order to preserve its integrity, so partnering with a vendor that has a ready-made library of customizable dashboards and pipelines helps eliminate this potential for setbacks.
6. Applications and integrations
Applications and integrations can be major difference-makers in the decision of which data platform works best for your organization’s needs. This category encompasses things like point-of-care integration with physician workflows, patient outreach (like a text messaging program), and care management workflows.
Essential? It depends. But something key to consider here is that streamlined applications will have a major impact on engagement. Getting providers to use a clunky, antiquated portal might yield little interest, whereas onboarding them with something efficient and intuitive could improve everything from reporting to morale.
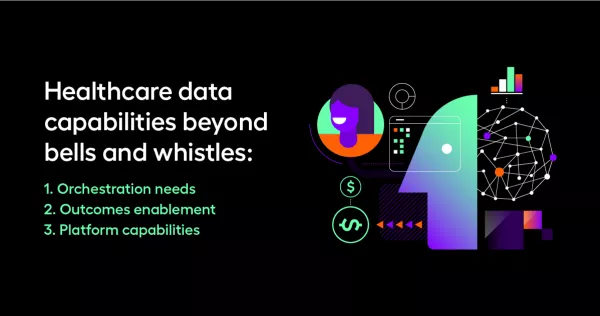
Healthcare data capabilities beyond bells and whistles
Knowing the key functions a data platform performs in healthcare empowers you to dig deeper when it’s time to pick a solution. With a sea of choices as vast as a data lake, it can be overwhelming, but a core understanding of the essentials means an informed, thoughtful choice.
Beyond core components, consider these necessary capabilities:
1. Orchestration needs
A great data analytics solution will pull data from numerous sources, and much can go wrong in that attempt to herd information. Data orchestration connects the storage systems an organization uses so that everything flows smoothly, and an ideal solution will help organize the data points flowing in and place them where they need to be accessed (like a provider portal or records system). This opens up the potential for security threats, though, so in the quest for the right solution, consider potential threats alongside things like interoperability.
2. Outcomes enablement
Similar to reports and business intelligence in the key components section, it’s essential that a healthcare data analytics platform can help drive the outcomes specific to an organization and its goals. It starts with getting clear on just what those are (whether it’s outstanding performance in risk-based contracts or reducing the A1Cs of a large diabetic population). From there, administrators can zero in on the reports, dashboards, point-of-care integrations, patient outreach tools, and other workflow tools or integration points that will matter most. These necessary tools will then dictate which data sources to integrate, and how that data will need to be contextualized or enriched.
3. Platform capabilities
What’s under the hood of a given data platform? A third-party solution could come with its fair share of weaknesses, but they can also be inadvertently built into a customized, in-house solution. Any data platform worth its bytes will offer a master patient index, provider attribution, data enrichment, and many other important data processing steps. The question is how well these functions perform, and whether one or two matter more to a particular organization than the others. If so, these can be lodestars that guide research and ultimately inform a decision.
Investigate options and hone in on what matters most
When you’re very familiar with what a healthcare platform can do, then you’re poised to figure out what it needs to do on behalf of your organization and goals. No two healthcare organizations are identical, so it follows that what they need in a data analytics platform will vary too.
Before browsing the market of available data solutions, before talking to vendors, before interviewing candidates for an in-house team, there’s a crucial first step: reflecting on your organization’s key goals.
Knowing exactly what needs to be achieved (and on what timeline) is a surefire way to eliminate unnecessary contenders and focus on a few suitable candidates. Whether that’s a crew of in-house employees with a suite of offices or an industry-leading tech company, whatever solution you choose should specialize in should excel where it counts for your most meaningful use cases.