Reducing implicit bias in predictive healthcare analytics
Machine learning and artificial intelligence can lead to healthier patients — don’t let implicit bias derail your efforts.
The most dangerous enemy is the one you can’t see. In predictive analytics, that proves especially true. When algorithms and machine learning focus on an outcome of interest, the results can impact innumerable lives — especially when that technology gets implemented in healthcare.
Here we share learnings from our recent white paper on some of the not-so-hidden dangers of implicit bias in medicine, plus best practices for eradicating it from your workflows. Learn from other data analysts’ mistakes so that, in the future, predictive analytics in healthcare reflect a more equitable field, in a more equitable world.
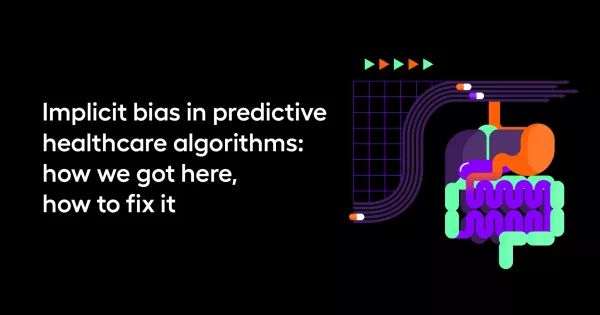
Implicit bias in predictive healthcare algorithms: how we got here, how to fix it
Firstly, it’s important to define implicit bias. If a bias is a general prejudice, implicit ones are the more insidious cousin, often subconscious or unspoken. An implicit bias means a tendency to use pre-existing knowledge of patterns and types to validate and characterize new information, even when those patterns are rooted in biased social or cultural perceptions.
It’s helpful to use concrete examples. A bias might be “Women are dramatic.” An implicit bias would take it a step further, with an implied addition: “Women are dramatic… so be careful when it comes to treating their pain.”
When an implicit bias exists in one human, it’s a problem. When it exists in an algorithm, it’s a danger. The trouble, then, is that unemotional, unbiased machines are programmed by imperfect people.
Predictive tools use the same mechanisms an individual person does: detecting trends and patterns to try to determine outcomes and outputs. People do this in harmless ways all the time — looking into a room and deciding whether or not it’s too crowded based on an initial peek, or seeing the line at a bagel shop and guessing the poppyseed will be sold out by the time it’s your turn.
Other times, though, the consequences are more dire — boiling a certain race down to “essential” traits, or making inferences based on appearance or income. Data analysts and programmers might be blind to their own biases, and if they’re coding a predictive algorithm, these prejudices can get baked into a system-wide platform.
To avoid this entirely, the first step is figuring out when predictive healthcare modeling makes sense (and when the problem in question does or doesn’t match the tools at hand).
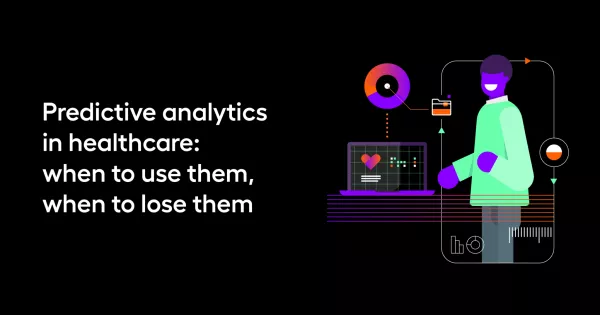
Predictive analytics in healthcare: when to use them, when to lose them
Before programming, before deep learning, before any intervention — it’s necessary to start at square one. Define the problem of interest. From there, additional questions crop up: what kind of data is at your disposal, and can that help you solve this query in particular?
If the answers look promising, five additional criteria prove key:
- The outcome isn’t a question of fact (or known fact) — If you can deduce the answer you want by simply poring over existing data, predictive analytics aren’t the right tool for this project. Even if the information is difficult to access, there are mechanisms that can help unearth it, but machine learning should only be applied when the facts aren’t known or discoverable.
- The outcome is quantifiable (or can be clearly defined) — This could look a yes/no question, or a number a team is targeting, like “What percentage of Type I diabetics subsequently develop a thyroid condition?” or “Does eating an apple every day keep patients out of their provider’s office at a statistically significant rate?” The definition of what the outcome looks like should be clear. Likewise, results need to be consistent, objective, and repeatable.
- The outcome, if known, would influence clinical decisions — If the resulting discoveries won’t shape the way patients are treated, why bother? The outcome should positively impact the field, even if that’s incremental. Researchers should have a meaningful reason for undertaking predictive work. Thinking about how this might manifest in a clinical setting might help clarify points 1 and 2 — what questions could be asked that would enable discovery, or establish new benchmarks?
- The outcome can be estimated for large groups of people — Avoid very small samples, much less individual cases. It’s critical as a statistician and data scientist to use larger groups for making an accurate determination, but it’s also important on the healthcare side (treating the largest swath of people).
- The consequences of the wrong choice are known and acceptable — What’s the potential outcome of applying predictive analytics, and do you understand what the risk is? Akin to #4, is there potential to do great harm, or misguide a large group? This becomes increasingly critical with terminal or chronic illness, or treatment decisions that materially impact a patient’s finances, lifestyle, or mental health.
If (and only if) this criteria suits the research at hand, machine learning and artificial intelligence have the potential to unearth insight and innovative care. Once these questions are answered, it’s time to look at different types of applicable models, potential pitfalls, and performance.
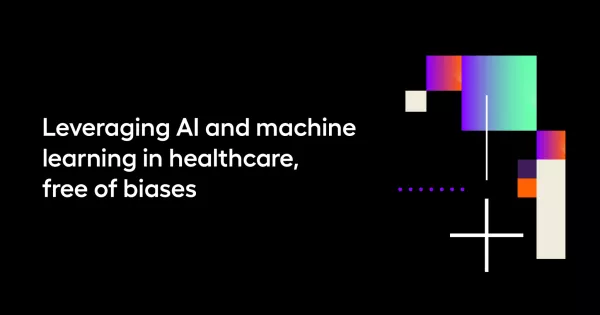
Leveraging AI and machine learning in healthcare, free of biases
With this in mind, how do you prevent implicit bias?
1. Define the affected population and use rich, longitudinal data to match.
If you’re designing an algorithm to make a prediction around a Medicare population, your data-set should be Medicare-based, with sufficient length and richness to characterize that group. You also want to make sure it matches or aligns with the demographics represented in that group — is it an appropriate characterization of Medicare population? The population you’re using to train the algorithm should be reflective of the population it’ll impact.
2. Select model outcomes that are universally accessible and applicable OR unavoidable.
The data could be correct within a certain model, but the outcome can still emerge biased, leading to biased care. One way around this is choosing predictive outcomes that are less prone to inherent bias, like avoidable ED visits or unplanned inpatient admissions, even if the chain of events that led to these happenings might be intwined with social determinants of health. Similar logic holds true for tracking negative outcomes, like patients with high A1C levels — there are lots of reasons why someone might not have access to care, nutritional meals, and other preventive measures, so changing the targeted outcome helps mitigate bias. Consider, instead, identifying the behaviors that drive well-controlled glucose levels, which can help providers implement them in larger populations.
3. Apply a critical eye to algorithmic outputs.
For example: of those that are referred to a care management program, or predicted to have an actionable outcome, are the participants suggested by the algorithm roughly representative of the full group? Those referred to a care management program might not be an exact racial and ethnic duplicate of the full population considered, but if it’s an overwhelming sample of one gender, race, or income level, something might be amiss. The resulting group should be roughly representative of the initial pool.
Where human fallibility can torpedo your AI’s integrity, it can also redeem it. Critical, thoughtful reflection will catch the important factors and considerations a machine misses.
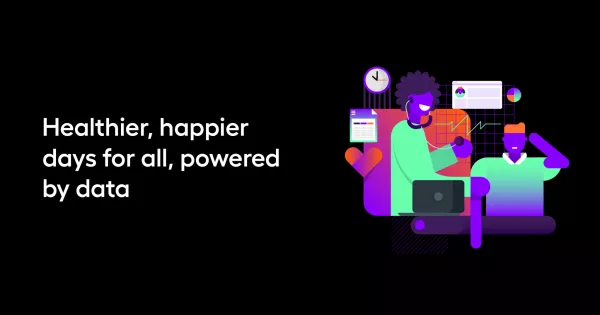
Healthier, happier days for all, powered by data
Arcadia’s credo means constantly striving for equity, inclusion, and data quality, all of which are inextricably linked. For more information on how you can leverage machine learning and artificial intelligence with an eye to objectivity, download our white paper, or drop us a line to find out how we can empower your system to provide better care.