A complete overview of predictive analytics in healthcare
Predictive analytics is revolutionizing many fields, and healthcare is no exception. It’s a key component for success across teams, enhancing patient care and improving operational efficiency for data-driven organizations.
In this guide, we’ll explore the role of predictive analytics in healthcare, including its benefits for different stakeholders and solutions for possible challenges.
What is predictive analytics in healthcare?
Predictive analytics is the evaluation of current and historical data through statistical modeling, data mining, artificial intelligence (AI), and machine learning techniques to predict future events.
It applies to many types of healthcare data, including structured data, like electronic health records (EHRs), and unstructured data, such as clinical notes and imaging reports. It also leverages big data to provide insights into patient care, resource management, and health outcomes.
IT operators, care managers, and other healthcare professionals use predictive analytics to surface insights and enable operational efficiencies.
What is predictive modeling?
Predictive modeling is the process of running mathematical algorithms on data to predict what’s most likely to happen in the future.
Although the terms are often used interchangeably, predictive modeling is considered a subset of predictive analytics. Predictive modeling describes the process of building a statistical model, while predictive analytics refers to the entire process of using data to make predictions.
How predictive analytics works in healthcare
The journey of predictive analytics in healthcare involves four stages:
- Data collection: This involves gathering information from various sources, including proprietary structured and unstructured data, public health records, and cutting-edge technologies like wearable devices.
- Preprocessing: In this stage, data undergoes cleaning to ensure accuracy and relevancy.
- Modeling: This stage uses data algorithms to make predictions and recommendations for specific patient populations.
- Interpretation of results: Finally, healthcare predictive analytics empowers you to move forward with strategic decision-making and policy formulation.
These stages empower users, whether they’re on the front lines of care or analyzing data in an office. For example, a provider may reference a list of helpful questions for the at-risk pre-diabetic patient sitting in front of them. A data scientist may build a model that transforms care delivery in a hospital’s oncology unit. In fact, many teams across the care continuum can benefit from predictive analytics.
How does predictive analytics benefit health teams?
We’ve broken down the benefits of predictive analytics in healthcare by team:
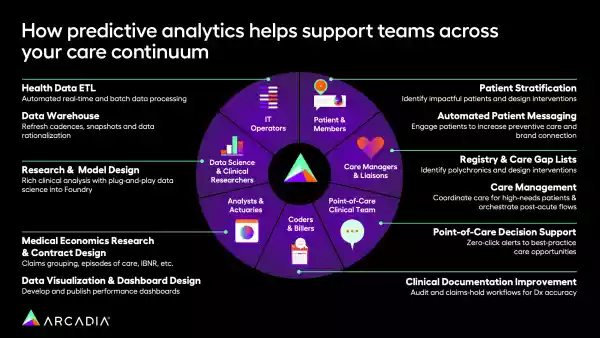
1. Patients and members
Predictive analytics is pivotal in revolutionizing patient care, helping patients get the right care at the right time. It serves the following key functions that benefit patients and members, among others:
- Patient stratification: Predictive analytics enables early diagnosis and preventive care by identifying health risks before they escalate. For chronic condition management, these analytics are indispensable, especially considering that chronic diseases, such as heart disease and cancer, are the leading causes of death and disability in the U.S. Care managers and clinical teams can use predictive models to create treatment plans tailored to individual patient profiles.
- Automated patient messaging: Predictive analytics enables automated messaging tailored to the specific audience that will receive it. By crafting and sending thoughtful, dynamic content, healthcare teams can promote preventive care and engage patients so they’re more likely to receive continuous, regular care.
Additionally, healthcare predictive analytics powers early reminders and helps providers encourage preventive care (screenings or vaccinations). Offering proactive approaches that equip patients and providers to work together toward preventive care ultimately results in improved health outcomes.
2. Care managers and liaisons
Care managers use predictive analytics to allocate time and resources where needed most in two critical ways:
- Registry and care gap lists: Predictive analytics helps identify polychronic patients (those with three or more chronic diseases), immediately surfacing insights so healthcare organizations can offer additional care.
- Care management: With predictive analytics, care managers can pinpoint high-need patients and coordinate care by focusing on the most intensive care management interventions. Additionally, predictive models enable care managers to orchestrate post-acute flows by monitoring patients’ progress and intervening proactively if needed.
Using predictive analytics to allocate resources enhances patient engagement and satisfaction by ensuring care is timely and efficient. By using predictive analytics to stratify risk, care managers can better target interventions, significantly improving outcomes.
3. Point-of-care clinical teams
Clinicians face immense pressure to choose the right action or diagnosis amidst a sea of choices and possibilities. Predictive analytics eases some of this burden by providing:
- Point-of-care decision support: For clinical teams, predictive analytics augments diagnostics with advanced machine-learning algorithms and proves invaluable in managing emergencies through real-time data analysis.
4. Coders and billers
Predictive analytics helps coders and billers refine processes to recoup wasted time and create lean, efficient workflows thanks to:
- Clinical documentation improvement: Predictive analytics helps measure risk, quality, and fraud. With the right tools, coders and billers can zero in on the information that matters most to document patients’ risk.
Predictive analytics also plays a crucial role in fraud detection and billing error risk assessment. Moreover, it ensures adherence to regulatory standards, thereby elevating the quality of healthcare services.
5. Analysts and actuaries
Analysts and actuaries benefit from the ability to surface insights throughout their organization and plan for possible outcomes. This helps them ensure sustainable performance in the following ways:
- Medical economics research and contract design: Predictive analytics serves as a compass for long-term strategic planning for analysts and actuaries. They can leverage it to assess financial risks and sustainability, ensuring a balanced approach to healthcare financing.
- Data visualization and dashboard design: Analysts and actuaries can leverage predictive analytics to distill their valuable insights into shareable images and dashboards so that other stakeholders can better understand performance and goals.
By harnessing predictive insights, analysts and actuaries can translate data into actionable information that drives informed decision-making.
6. Data scientists and clinical researchers
For data scientists and clinical researchers, predictive analytics is key to new discoveries. It enables more thoughtful studies and sharper insights around central questions. Your team can achieve these insights with:
- Research and model design: Predictive analytics bolsters the development of breakthrough treatments and drugs. Data mining and pattern recognition, essential for drug development and clinical trials, heavily rely on predictive analytics.
7. IT operators
IT operators empower their organizations with predictive analytics by building the infrastructure for accessible, trustworthy data through:
- Health data ETL: IT operators use predictive analytics to maintain data quality and security. Predictive analytics helps IT operators extract, transform, and load data safely and with integrity.
- Data warehouse: Great infrastructure and data governance are essential for predictive analytics to work properly. A data lakehouse allows an organization to access its data in many forms, whether that’s unstructured and raw (for a data analyst) or very refined (for a provider at the point of care). Features like native deletions and offset management mean that users of predictive analytics don’t need to worry about an invalid data point spoiling the accuracy of a dataset.
In structuring and organizing an organization’s data properly, IT operators expand other users’ predictive analytics capabilities with reliable, vetted information.
5 uses of predictive analytics in healthcare

1. Readmission prevention
With nearly one-in-five adult patients experiencing hospital readmission, healthcare providers must proactively address patients’ follow-up needs with personalized discharge protocols. Using healthcare predictive analytics, providers can identify patients at risk of readmission and allocate resources to prevent such a turnaround.
Not only does this prevention benefit the patient, but it also lowers the U.S. healthcare system’s spending on readmissions, which is currently around $52.4 billion per year. It’s also beneficial to the 82% of hospitals penalized under Medicare’s Hospital Readmission Reduction Program (HRRP).
2. Clinical decision support
Predictive analytics unlocks the ability to anticipate patient outcomes, optimize treatment plans, and improve overall care quality. When applying predictive analytics, clinicians can:
- Diagnose conditions early: Identify early signs of diseases that may be difficult to detect in the initial stages
- Select treatments: Forecast a patient’s response to specific treatments, minimizing the need for trial-and-error approaches
- Monitor critical conditions: Analyze real-time patient data to spot early warning signs and improve patient survival rates
Equipped with the full context of a patient’s needs, clinicians can make informed decisions with confidence in positive patient outcomes.
3. Health insurance management
As healthcare predictive analytics provides insights into the factors that influence care utilization and costs, this information gives health insurance providers a deeper understanding of:
- Utilization: Providers can proactively meet care needs and mitigate unnecessary costs through predictive analytics. This allows them to accurately forecast a patient’s healthcare utilization, such as hospital admissions or surgeries, based on historical data and clinical variables.
- Cost prediction: A patient’s expected healthcare costs can be forecast by understanding their past utilization and treatment needs
- Fraud prevention: Payers can analyze claims data and identify fraudulent behavior patterns to detect it early and prevent insurance abuse. This would significantly impact the financial side of patient care, since financial losses due to healthcare fraud account for 3% to 10% of funds spent on healthcare, or $300 billion.
Calculating the accurate cost of insurance empowers patients to receive the care they need without financial hindrances.
4. Population health management
Providers can take patient data beyond individual care to identify cohorts with shared medical characteristics and prevent population-wide health risks. For example, providers may be able to detect disease outbreaks early by using predictive analytics to identify groups with potential exposure. As a result, providers can improve population health management efforts via timely treatment development and plans.
5. Clinical research
90% of drugs fail clinical trials. However, research suggests there are opportunities for artificial intelligence to alleviate the issues behind these failures. Using predictive analytics, AI can:
- Identify eligible patients
- Enroll those who are likely to participate
- Determine appropriate sample sizes
- Reduce inconsistencies in protocol
- Improve the use of funds
By addressing these issues, the technology behind predictive analytics enables researchers to conduct trials more efficiently, increasing the likelihood of reaching groundbreaking results.
Umpqua Health: An example of predictive analytics in healthcare
Umpqua Health is a CCO serving 35,000 Oregon Health Plan Medicaid members in Douglas County. In response to recent wildfires consuming more than 100,000 acres in the state, Umpqua distributed air purifiers to members affected by the poor air quality from wildfire smoke.
However, people with respiratory issues are more at risk of being harmed by the smoke from these fires. In anticipation of the upcoming wildfire season, Umpqua wanted to proactively distribute air purifiers to those who needed them.
Enter predictive analytics. Using Arcadia’s platform to analyze SDoH data, past events, and claims data, Umpqua predicted individuals’ future risk for respiratory complications.
“Once identified, we use Arcadia’s functionality to notify members through a text that they qualify to receive this device and how to get it. The devices were then distributed through our transitional care clinic to our members and we have our community health workers delivering some to our homebound members.”
Applying predictive analytics to their data enabled this organization to identify at-risk patients and proactively address their needs, preventing costly health issues and interventions.
Challenges and ethical considerations
Despite its many benefits, predictive analytics in healthcare has risks and challenges. Some top concerns include:
- Data security and privacy: Given the sensitive nature of health information, healthcare data is a huge target for cybersecurity issues. To maintain patient trust, comply with HIPAA regulations, and protect human privacy, providers must employ strict data security measures.
- Accuracy and reliability: Predictive models are under constant scrutiny to avoid potential misdiagnoses, biases, or treatment errors. This leads to a lack of trust in the model.
- Provider acceptance: Providers must balance the need for enhanced patient care through data collection with the time and effort required to leverage analytics. From training on new technologies to implementing new workflows, the process may involve significant changes for providers.
This doesn’t necessarily spell doom for predictive analytics in healthcare. By enforcing data access controls and obtaining consent before sharing data, providers can benefit from predictive analytics while still protecting patient privacy. Still, it does mean that users need to approach any technology with a clear sense of when it’s appropriate, how to avoid missteps, and how to read results with a critical eye.
Ethical considerations, such as addressing healthcare disparities and mitigating bias in data and algorithms, also need ongoing attention. For example, implicit biases can find their way into predictive analysis algorithms, leading to unfair outcomes. You’ll need to critically examine algorithmic outputs to eliminate any biases.
The future of predictive analytics in healthcare
Predictive analytics impacts every facet of healthcare and opens up exciting possibilities, from earlier interventions to a more customized, thoughtful, and engaging patient experience. As a powerful tool for enhancing healthcare delivery, it offers insights that are instrumental in driving efficiency, accuracy, and improved patient outcomes.
The future trajectory of predictive analytics in healthcare is both promising and challenging. Emerging technologies like AI significantly augment predictive analytics capabilities, and healthcare providers must embrace this technology to stay at the forefront of healthcare innovation.
Success in predictive analytics requires the right foundation, and a sophisticated architecture (like a lakehouse) is the first step in leveraging this technology. The integration of these technologies into healthcare workflows is vital for realizing their full potential and improving patient outcomes and operational efficiencies.
With the right mix of thoughtfulness and innovation, technology advocates and healthcare pioneers can collaborate toward a more predictive and proactive healthcare system.