3 ways to reduce bias in predictive analytics for better health equity
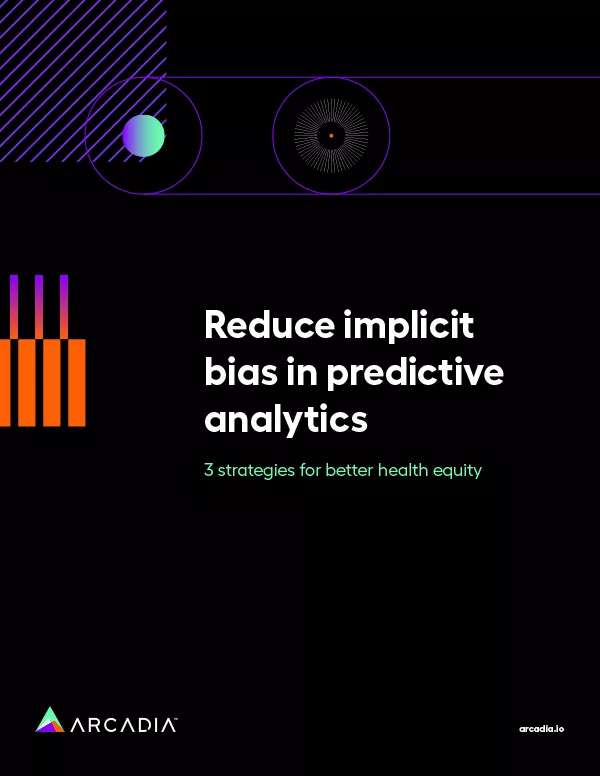
Implicit bias and inequities can easily get baked into Artificial Intelligence (AI) and predictive tools without an intentional approach to development that specifically addresses this issue.
Here, we offer an approach that highlights the importance of bias mitigation and consideration of health equity issues in AI tool development, as well as several tips for reducing implicit bias and improving the equity and diversity of predictive outputs while still optimizing the organizational and financial objectives of their design.
This white paper is targeted toward practitioners and developers of data science looking to improve their approach toward bias mitigation, as well as clinicians and analysts who want to understand the ethical and equity implications of using predictive tools in the health care and research arena.
Author: Michael A. Simon, Ph.D., is the Director of Data Science at Arcadia, the leading population health management and health intelligence platform. He was the principal researcher of the study, Reduced Incidence of Long-COVID Symptoms Related to Administration of COVID-19 Vaccines Both Before COVID-19 Diagnosis and Up to 12 Weeks After, available on medRxiv. He also contributed to a white paper on that topic, What Drives Long-COVID?.
COVID-19 brought a focus to health inequities
The COVID-19 pandemic has brought into sharp focus the racioethnic and socioeconomic disparities inherent in the U.S. healthcare system. These disparities take the form of increased adverse health outcomes as well as reduced quality-of-life for affected groups.
For example, a study of cities that reported COVID-19 deaths by race and ethnicity found that 34% of deaths were among non-Hispanic Black people, a group that accounts for just 12% of the total U.S. population, according to the U.S. Centers of Disease Control and Prevention (CDC), citing “long-standing systemic health and social inequities” among the reasons for the racial and ethnic disparities in COVID-19 deaths.
This heightened awareness around inequities and disparities in healthcare has also resulted in some much-needed attention to similar bias-related problems in the growing sector of Healthcare AI. As an increasing number of healthcare organizations have the opportunity to exploit more comprehensive data and improved predictive tools to improve their patient risk identification and clinical decision-making, they also become more susceptible to the effects of implicit bias, a natural consequence of intelligence that can, if unchecked, exacerbate the very health inequities that they were designed to relieve.
Why implicit bias in data science matters
Implicit bias, or the tendency to pass through thoughts confirming or conforming to stereotypes, is a natural condition of human cognition. Perhaps most famously articulated by the Harvard Implicit Association Test, implicit bias reflects our tendency to use pre-existing knowledge of patterns and types to validate and characterize new information, even when those patterns are rooted in biased social or cultural perceptions.
It shouldn’t be surprising that artificial intelligence is subject to the same kinds of implicit biases. After all, predictive algorithms and machine learning tools are ultimately advanced pattern matching systems, similar to how our own minds attempt to derive insights through patterns.
This connection was made more concrete in a study by Ziad Obermayer and colleagues published in the journal Science (DOI: 10.1126/science.aax2342) in 2019. Obermeyer’s team studied a predictive algorithm widely used by healthcare organizations to stratify patients by risk of future health needs for potential care management enrollment. Such tools help organizations identify which patients should be targeted for additional care. However, since assessing a patient’s “future health needs” is complicated, the developers used cost as a proxy for need, a not uncommon way to estimate future complexity of care.
The problem with this approach is that spending patterns differ substantially by race, ethnicity, and socioeconomic status. Using machine learning to train a tool to identify need based on cost resulted in consistent underestimates of the future needs of Black patients. Since access to care may rely on algorithms like this, implicit bias in those outputs can make it more difficult for these patients to obtain care. Researchers reported that they found evidence of “racial bias” in the algorithm output that translated into a reduction by more than half of the number of Black patients identified for extra care.
Improving the state of healthcare AI is possible
Studies like Obermeyer’s are a wake-up call, not only for data scientists but also for clinicians and analysts in the healthcare industry, both because of the increasing use of predictive and suggestive tools in healthcare as well as the pernicious way that implicit bias and drivers of healthcare inequity can seep into their designs, even when created with the best of intentions.
The good news is that bias and inequity in healthcare AI are not inevitable. Just as an individual’s implicit biases can be countered through intentional thought and action, implicit biases in algorithmic and model design can be countered through intentional design and training. The objective is not the elimination of all biases — that’s no more possible than elimination of all error in a model — but rather the mitigation of implicit biases and validation of outcome parity.
Ask yourself these questions: is this healthcare problem appropriate for predictive analytics?
One place to start is by considering when it is and is not appropriate to use predictive analytics models. For example, we apply the following five criteria when deciding whether a problem is appropriate for predictive analytics:
- The outcome isn’t a question of fact (or at least, a known fact) — put another way, if there’s a way to find out the answer to the question based on what is known, do that.
- The outcome is quantifiable or, at least, can be clearly defined — if the question to be answered can’t be measured, would trying to “calculate” it make much more sense?
- The outcome, if known, would influence clinical decisions — if the answer wouldn’t help provide better and more equitable care, maybe we’re asking the wrong question.
- The outcome can be estimated for large groups of people — applying models to extremely rare scenarios may not be worth the error rates impacting those most affected.
- The consequences of the wrong choice are known and acceptable — no model is perfect; can the result of an erroneous action (or inaction) be justified for one person? For a thousand?
With these criteria in mind, there is a vast universe of appropriate and beneficial applications of AI in healthcare. Given these opportunities, both for patients and their providers, how can we best mitigate the effects of implicit bias?
Diminishing implicit bias in 3 actionable steps
By their nature, predictive algorithms bring the possibility of perpetuating old biases or perhaps even introducing new ones into clinical and population health decision-making. However, by keeping the following three principles in mind, data scientists can lessen bias and promote greater health equity in the predictive algorithms they develop:
- Define the affected population and use rich, longitudinal data to match.
- Select model outcomes that are universally accessible and applicable or unavoidable.
- Apply a critical eye to algorithmic outputs.
1. Define the affected population and use rich, longitudinal data to match
Predictive algorithms can help clinicians make better, more cost-effective decisions more quickly, but they must be based on data that represent the targeted patient population. Ideally, models would be trained on an extremely rich dataset with broad ethnographic coverage, including race, ethnicity, sex, geography, and socioeconomic status.
Widely used electronic health records and analytic tools such as synthetic controls have helped to expand the availability of such data. This means that data science teams can more easily develop tools that are appropriate for the patient populations they serve.
For example, a model intended to predict progression of chronic kidney disease could allow providers to offer interventions before progression becomes too severe. Access to a broad training dataset with diversity among demographic and socioeconomic groups would allow the development of a model that can make predictions for a diverse array of patients.
However, access to such a broad-reaching and diverse dataset is not always possible. When this is not an option, ask whether the scope of the model can be fairly redefined to the available data. For example, if the data become limited outside of a specific Medicare population, it may be prudent to create a model for that group, and then test applicability to other groups. While we want to expand services to a diverse array of patients, attempting to do so using a model that will be biased against vulnerable and underserved populations is arguably worse than no model at all.
2. Select model outcomes that are universally accessible and applicable or unavoidable
One of the most important outcomes of Obermeyer’s analysis was that once a model has been trained on a biased outcome, a biased result is inevitable. For example, total cost of care is often chosen as a proxy for adverse health outcomes (and a direct signal of likely ROI). Since historical cost of care is one of the best predictors of future cost of care, historical cost can easily become a primary driver for deciding which patients get additional care. It also potentially overlooks a broad set of individuals who use (or don’t use) the healthcare system in a “traditional” or normative way.
In essence, the model “learns” that patients who now or in the past have experienced a lack of access to care, a lack of referrals to appropriate providers and services, and other social and cultural barriers to care are less desirable targets for intervention. This is not only inaccurate, resulting in poorer model performance, but also directly biases interventions away from patients of color, and particularly Black patients, as well as other underrepresented and vulnerable populations, including female, indigenous, LGBTQ, and homeless patients.
In contrast, model outcomes that are broadly accessible as well as applicable or unavoidable reduce the likelihood of learned implicit bias. A model trained to predict unplanned or emergent inpatient events covers a much broader group of individuals than one trained on all inpatient admits (including costly elective surgeries) and has the added benefit that that the events being predicted may be better impacted by an intervention.
Positive or desirable outcomes, such as medication adherence or preventive care, can also be effective as model training targets, provided the objective is to steer all patients toward that outcome and the intervention ensures that all those patients have access.
3. Apply a critical eye to algorithmic outputs
You can’t undo model bias by tweaking the outputs to make it “fairer”, but you can make output bias and equity one of the focus areas in your model validation process.
Ethnographic parity is an easy start; if the proportions of different racial, ethnic, and other demographic groups are wildly different in model outputs compared to your patient population, it’s not unreasonable to pause and ask why. It doesn’t mean such differences are “wrong” (or that perfect parity would be “right”), but it does prompt the question of whether strong differences have a biological or operational reason, as opposed to a source bias.
Evaluation of outcome diversity based on conditions and resources can also be valuable touch points. If an outcome involves utilization of a healthcare resource, could barriers to access or assumptions about need be biasing results? For example, a metastudy of heart failure treatment and outcomes showed both less frequent provision of care as well as poorer outcomes for people of color, women, and Black women in particular. If a universally applicable and accessible outcome is deferred due to biased human assumptions about pain and appropriateness, model outputs may encourage similar results based on learned, biased algorithmic assumptions.
Design for effective, actionable, equitable predictive tools and programs from the start
Crafting model outcomes that support equitable access to care is no easy task. In the model development lifecycle, we find that it is the most sensitive and deliberate step of the entire process — which is why we make sure it’s among the first steps of the process. We seek out as much input as we can from as broad a base of clinical experts as possible.
For those making use of predictive models, designing target interventions and intake criteria that are effective, equitable, and straightforward to use is a sizeable challenge, as well. And, of course, no algorithm or model is 100% without bias — hence the need for continuous awareness and improvement.
Given the recent focus on inequities and disparities in the health system, healthcare data scientists and those who make use of their products must incorporate a bias mitigation strategy into their development process. With an intentional and thoughtful perspective on what makes predictive analytics useful, effective, and fair, there is a strong future for healthcare AI to change the way patients experience care (and the way providers deliver it) for the better.
Drive better clinical outcomes with massive, diverse, longitudinal real world data on the Arcadia Analytics platform
Arcadia’s purpose-built, population health platform enables our healthcare customers to consistently over perform industry average outcomes by reducing medical expense and improving quality, risk coding accuracy, and patient health outcomes. Arcadia’s platform continuously aggregates and curates the highest quality, most complete and up-to-date data foundation, provides relevant, timely, and predictive analytics, and enables action through care management tools and in-workflow insights that present at the point of care.
Arcadia Research Data is built on an actively growing clinical and financial data asset enabling improved innovation for your research teams. Increase time spent on high value analysis, drive efficiencies across datasets, and unlock insights with deeper clinical details across the development and regulatory continuum. Arcadia Research Data is drawn from a national patient population across all sites of care with comprehensive payer coverage ensuring unique visibility across formularies and the entire patient journey. Start the conversation to find out more.