A population under a microscope
The A population under a microscope: Measuring risk and impactibility data visualization concerns finding the demographic factors (including social determinants of health) that indicate potential for complex care management.
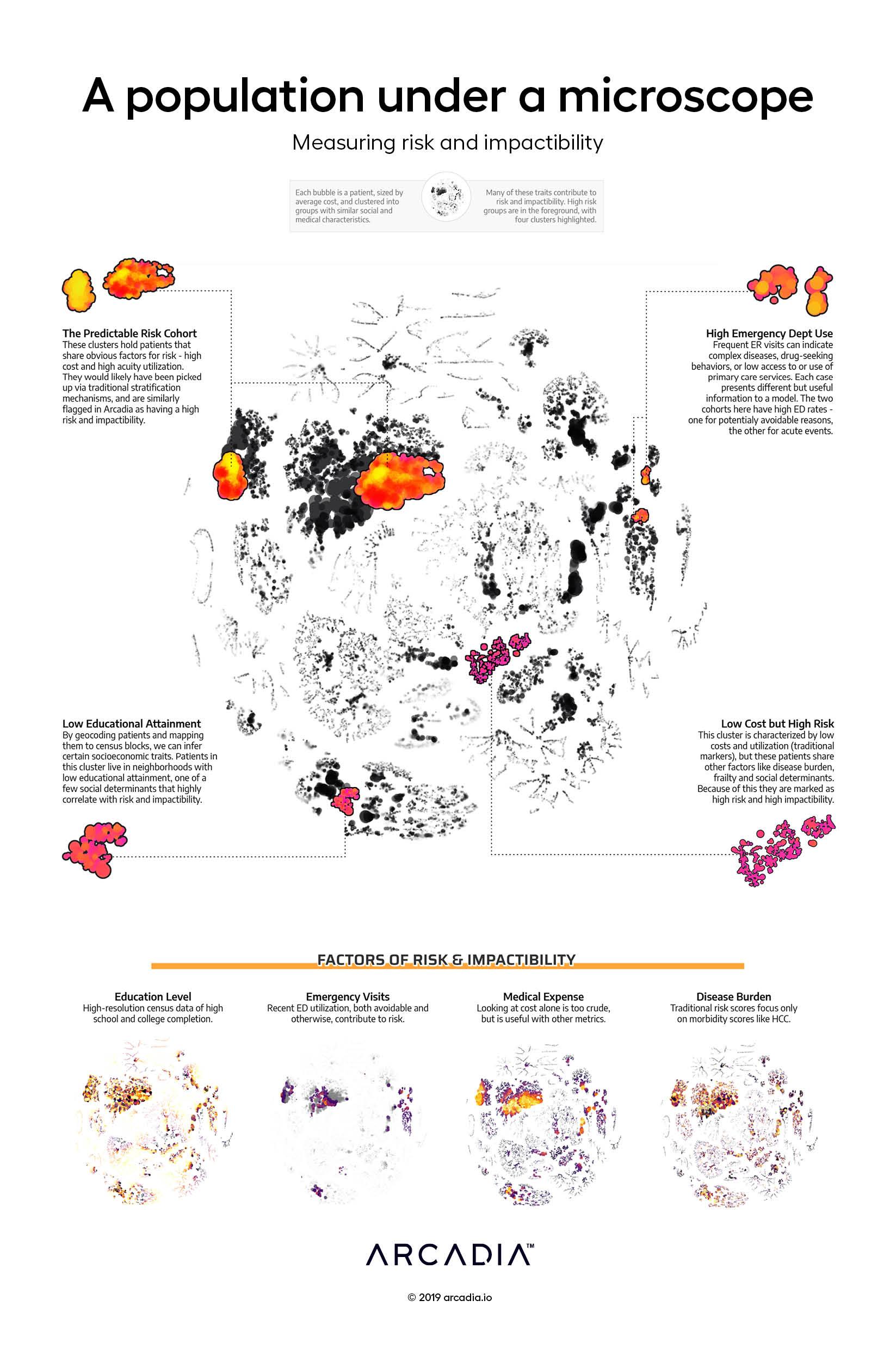
Care management programs are not one-size fits all. Rather, patients have subtle characteristics requiring tailored patient-centered plans of care. Similarly, the extent to which the patient will benefit from the targeted program varies as well. Identifying those who will be most successful in a given care program takes a combination of medical expertise, research, and mathematical estimation. Arcadia quantifies this “impactability” with learning algorithms that look through hundreds of thousands of past cases, finding shared attributes in those that had the greatest success.
This illustration analyzes about 64,000 patients and their predicted impactability (main diagram) along with their characteristics (sub-diagrams) to answer the question: what characteristics are the strongest indicators of success in complex care management?
Taking the population under a microscope, by applying t-SNE (a dimensionality reduction technique) and subsequently studying the resulting clusters, we find that four distinct groups of patients exist that have high impact scores, and many more small groups. While the largest group is composed of the most predictable at-risk patients (high cost and complex conditions; top left), the other groups show: 1) a unique pattern of avoidable and emergent Emergency Department utilization lead to high impact scores (top right), 2) a particular combination of chronic, psychosocial, and frailty conditions lead to high impact scores (bottom right), and 3) certain socioeconomic traits, along with a set of specific conditions, lead to high impact scores (bottom left).
Arcadia’s impactability algorithm consumes more than thirty specific risk factors — nearly impossible for care managers to keep track of over all patients, but easy for the algorithm to combine into one risk score tailored to care management. This algorithmic assist not only finds more actionable patients, but also lets program coordinators repurpose their valuable time from patient identification to patient engagement and program design.
Details
Python, D3.js, with Illustrator
Data sourced from Commercial, Medicare and Medicaid claims from Arcadia Benchmark Database