Are my data healthy?
When data’s informing critical healthcare decisions, it needs to be ironclad. But the journey from a data point to a refined piece of usable information isn’t always straightforward, and what’s more, it’s riddled with potential snags.
Here, Arcadia VP of Data Operations Mary Kuchenbrod explains how healthcare leaders and data experts can take the pulse of their data’s health, from the point of entry to its ideal use as a lever for better population health.
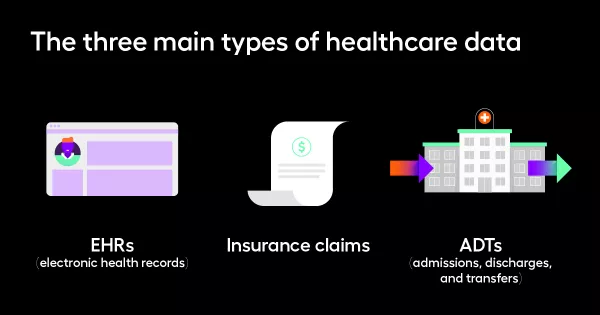
The three main types of healthcare data
In an analytics capacity, there are three main types of healthcare data, all of which come with their respective upsides and downsides. The first step in auditing your data’s health is recognizing what types of data you use.
1. EHRs (electronic health records)
This crucial data comes with a helpful sense of immediacy. It’s what’s recorded in doctor’s office at a visit, for example, so it has the potential to shed light on the most important parts of a patient’s health. But it’s often less structured, like a questionnaire or a dropdown menu that was selected at the beginning of the session, so integrating it within another data set can be a challenge.
2. Insurance claims
On the other end of the spectrum, the claims filed by insurance companies often arrive 90 or 100 days after a patient’s care, but they’re precisely structured. This data is introduced by the health plans during billing and adjudication, and while it won’t help you take immediate action, it’ll arrive complete and contextualized.
3. ADTs (admissions, discharges, and transfers)
This hospital workflow stores data around admissions, discharges, and transfers in an urgent setting. Think: a patient just checked into an emergency department. These are pieces of information that you want right away, no matter how unstructured, largely because they allow care management or outreach teams to reach out or intervene right away.
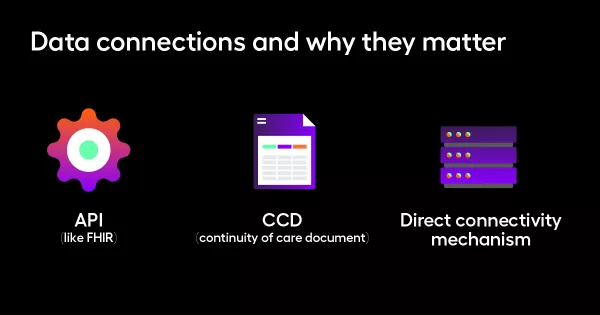
Let’s link: Data connections and why they matter
Once you’re familiar with the data types, looking into how your analytics platform connects to these sources is imperative. There are several common connection types:
1. API (like FHIR):
An API is a common language, allowing information systems to easily send and receive important data. The information flows rapidly, but there’s a risk of lost detail, so it’s ideal to augment this with another source.
2. CCD (continuity of care document):
A continuity of care document centralizes a patient’s clinical information digitally, described by this site as “comprehensive yet concise.” Like the API above, it’s a quick way to get the gist of a patient’s health, but it’s not comprehensive.
3. Direct connectivity mechanism:
Customizable in nature, direct connections with a data source offer the ability to calibrate a data feed to the level of preciseness you need. A data platform could connect to the database from whence their EHRs come, or just request periodic outbound flat files with a broader set of information.
Supplemental sources: Sprinkles on the data sundae
“What are you also going to need — in addition to the main core data sources that you have — in order to have the right types of data in the system?” Kuchenbrod asks. This is where the health of your data is specific to your use case: do you have enough reliable, contextualized information to make a particular type of decision within your organization?
This supplemental data can take many forms, but once again, it should be informed by the most pressing questions at the heart of your work. It could be external labs, for example, to help corroborate a diabetes care management program. It could be a scheduling interface feed, to know who’s on the books for an upcoming visit (and who’s not). The options are vast and ready to be tailored to your use case.
It’s time to think about data timing
Beyond considerations of source and connection, Kuchenbrod emphasizes that organizations need to keep an eye on the clock. Specifically, they must think about the timing of the data use.
The first question: when are you getting it, and is it aligned with when you intend to use it?
“I might get data daily, for example, but calculate quality measures once a week,” Kuchenbrod says, in which case a healthcare network could ensure the volume of that data isn’t too vast (and detailed) or paltry (and lacking in important specifics).
Other questions around timing include: What do you need to calculate with the data when you have it? What is the frequency with which you’re undertaking the data-driven process in question? How do you ensure that data over time is staying fresh, that you don’t have issues with data recency or lags? And finally, how do you monitor these facets over time?
“If you’re doing a pre-visit planning workflow, you need to be doing that right before the patient comes in for the visit,” Kuchenbrod says. “Whereas if you're running a monthly analytics report that you're going to show to your board or your medical directors, it's less important that that data have yesterday's care on it, and more important that you have time to vet that information and prepare a really useful analytic deliverable.”
Before you transform healthcare, note your data’s transformation
The final step in this equivalent to a data physical? A thorough look at data’s transformation from points A to B and beyond, noting how it’s changed as it progressed from user to user.
In the course of your data check-up, it’s important to ask: What happened on that data journey? Was it appropriate?
Can you look at the data now and know (correctly and accurately) what happened at the original point that care was delivered? Is it normalized to a common data model, so that it’s EHR agnostic for central utility?
Finally, how are you handling different levels of structure? Do you need NLP (Natural Language Processing) to get the most use out of it, or is the way the data’s currently structured serving your needs?
The healthcare data journey starts with a single step
Wherever you find yourself in your data journey, it’s not too late to refine this process and whip your information into usable shape.
If you find yourself at a crossroads (or just taking the first step), Kuchenbrod has this advice:
“I would say that an organization should start with a quick win, value-add use case, and understand what the speed and transformation requirement is for that specific use case,” she says. “If my first use case is calling patients when they get discharged from the hospital to make sure that they're getting directed to the right care pathways, then I probably value speed of information more than anything else, so I can know the same day that that patient was discharged, what happened, and take action. I don’t need to know much more data in that circumstance.”
Reach out to our team and learn how we can partner for optimal outcomes.