Healthcare data lake vs. warehouse: What’s the difference?
The pace of change in healthcare and technology can be dizzying, and for many healthcare organizations, keeping up means choosing the right data platform. There are so many options on the market that it can be overwhelming, but what’s important is that the one you choose enables your particular business goals and desired outcomes.
If change is the only constant, then healthcare organizations must equip themselves with adaptable, flexible tools, future-proofing their data architecture so they can embrace whatever the next wave of innovation brings.
Whether it’s machine learning (ML) or large language models, artificial intelligence (AI) makes a compelling case for data lakehouses — a combination of a healthcare data lake and warehouse that enables both structure and dexterity. Here, we’ll explore why data lakehouses matter in healthcare, and how different data storage models function.
What’s the difference between a healthcare data lake and a warehouse?
Before we discuss the data lakehouse, it’s important to break down its predecessors — the structures that inspired it — data lakes and data warehouses.
What’s a data lake in healthcare?
Think of a data lake as a vast, deep pool of data, an “ecosystem” of structured, unstructured, and semi-structured information all swimming around together. A data lake houses these diverse types of data because it can ingest data from many different kinds of systems, whether it’s on-site or off, and it can store a massive amount of this information securely.Healthcare data lakes are flexible, scalable, and primed for raw data storage. In this sense, their lack of structure can be a major asset, allowing for speed, volume, and depth.
What’s a data warehouse in healthcare?
Data warehouses differ from data lakes in important ways, but the two are often complementary. Where a data lake stores a mass of diverse data points of varying structures, a data warehouse focuses on analytics.
Imagine a big retailer’s robots fetching rows upon rows of boxes, then picture those aisles extending beyond your line of sight. Data warehouse systems are structured, meaning the data is more uniform and coherently organized than within a data lake. That makes it ideal for reports, analysis, and business insights.Its main strengths are its sturdy foundation, speed, and analytical capabilities. With the right underlying structure, a data warehouse can generate presentable analyses for business stakeholders, improve clinical decision-making, elevate strategic planning, and enhance outcomes.
What’s a data lakehouse?
Data lakehouses combine the vastness and flexibility of data lakes and the coherent organization of data warehouses in a hybrid approach to storing data for more comprehensive analytics. Bringing the best of both under one architecture, this technology is the latest and greatest solution in data storage and analytics.
As AI and machine learning emerge with more novel use cases, the data lakehouse is a structure that supports innovation, revolutionizing healthcare. With an additional layer of metadata and governance, it filters bad or unusable data but makes unstructured data available for analysis.
For a lakehouse to deliver useful insights and better efficiency, it needs to perform the following functions:
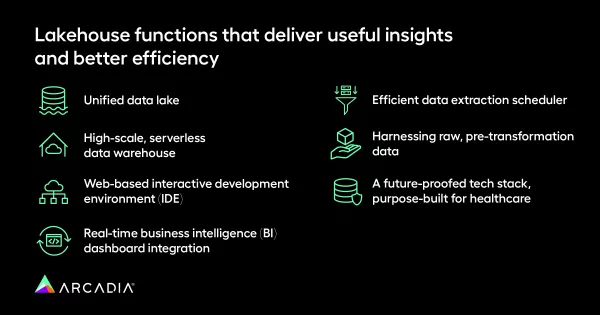
For a lakehouse to deliver useful insights and better efficiency, it needs to perform the following functions:
- Unified data lake: This is a reservoir of diverse health data, and it enables consistent ETL processes, advanced management capabilities, and seamless cloud integration
- High-scale, serverless data warehouse: This offers direct access to data via standard SQL interfaces, which allows healthcare organizations to build robust data security tailored to their needs
- Web-based interactive development environment (IDE): This provides direct querying capabilities, enhancing agility and decision-making speed
- Real-time business intelligence (BI) dashboard integration: This allows organizations to design intuitive reporting workflows that aid healthcare decisions and securely disseminate insights across departments
- Efficient data extraction scheduler: This allows for timely, automated data extraction tailored for healthcare operations
- Harnessing raw, pre-transformation data: Healthcare organizations need the flexibility to use vast arrays of external health data sources and aren’t constrained by the rigidity of a traditional storage system when they use a data lakehouse
- A future-proofed tech stack, purpose-built for healthcare: A data lakehouse needs to offer all of the aspects above, from data storage to analytics and extraction tools, but critically, it also must be ready for the future. It should bring scalability, security, interoperability, and cost-efficiency to an organization's workflows, and offer the ability to adapt as healthcare challenges and regulatory requirements evolve.
Simply put, the lakehouse outperforms the data warehouse and data lake, simpler models that preceded and inspired it. By offering the best of both worlds, the data lakehouse marks a meaningful evolution in healthcare and beyond.
3 reasons to switch from your data lake or warehouse to a data lakehouse
Sure, the technology that powers a data lakehouse is impressive, but in the end, what matters most is how it enables better healthcare performance. There are three key areas where data lakehouses drive enhanced care and outcomes, helping healthcare groups achieve efficiency in the process, especially compared to a data warehouse or data lake alone.
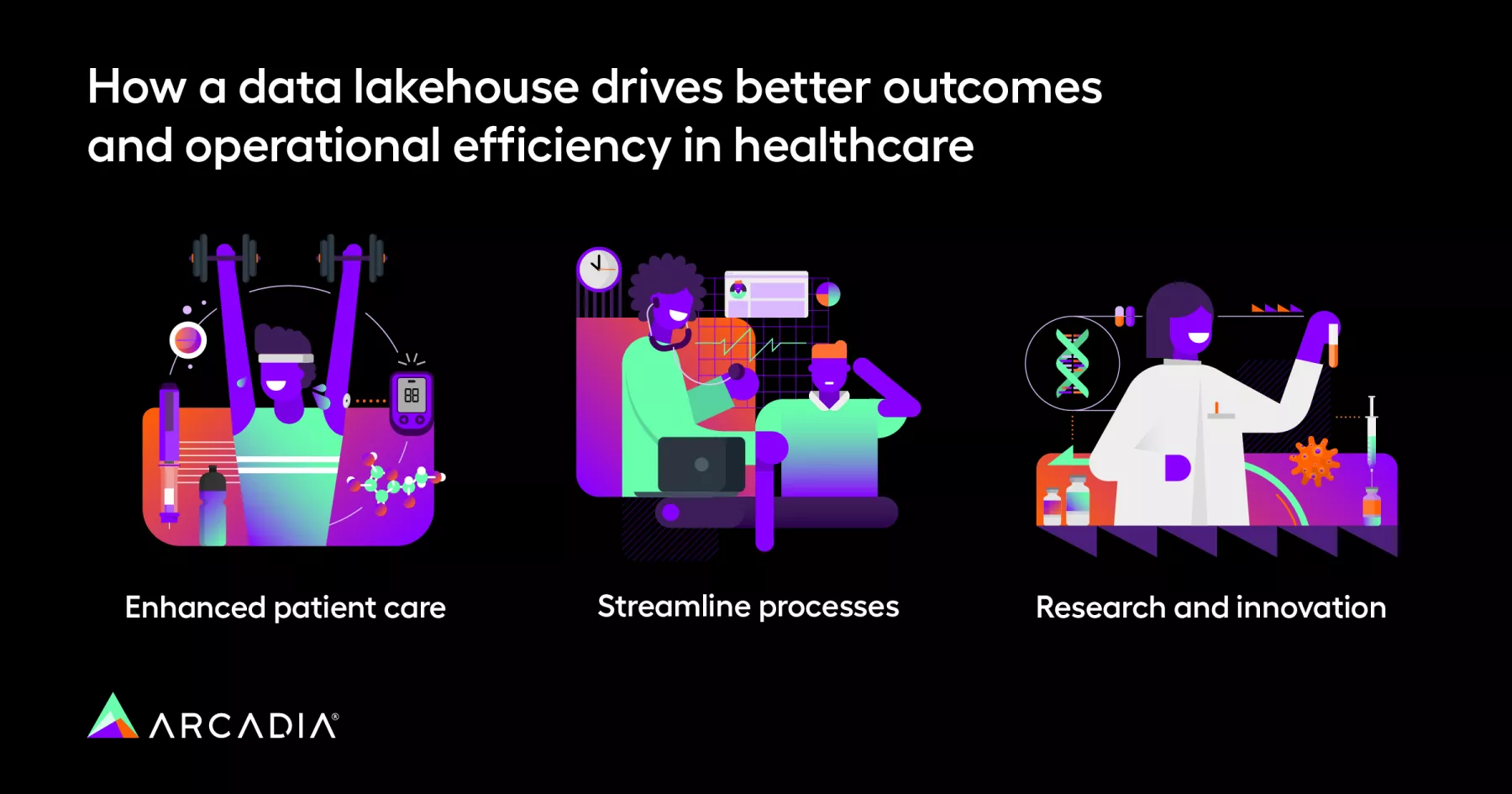
1. Enhanced patient care
With trustworthy, reliable, and timely data at your disposal, you can develop personalized treatment plans and implement effective preventive care strategies. Additionally, this data-driven approach facilitates proactive care by equipping providers to identify potential health issues early and address them promptly. As a result, patients will receive timely care tailored to their specific needs, improving overall outcomes.
2. Streamline processes
High-quality, relevant data improves operational efficiency for healthcare organizations by streamlining processes such as:
- Patient admissions: Accurate data improves the patient experience by simplifying the admission process and reducing wait times
- Treatment planning: Providers can make informed decisions about the course of a patient’s care with comprehensive and clean data
- Billing: Effective data management allows healthcare organizations to streamline billing processes, reducing administrative costs and enhancing overall financial performance
Better data management reduces unnecessary busywork, allowing providers to focus on patient care rather than administrative burdens. Additionally, streamlined processes can potentially prevent or reduce staff burnout.
3. Research and innovation
Comprehensive, real-time data access drives breakthroughs in medical science, empowering research and innovation. Data can accelerate the pace of research by providing the context needed to identify trends, correlations, and anomalies. Researchers can use this data to develop new treatments, improve patient care strategies, and more.
Evolve from the data lake and data warehouse to the best of both
If the future of healthcare is data, and the future of data is the lakehouse, it’s time to dive in. Data lakehouses are already transforming healthcare, enabling next-generation features in generative AI and ML.
A data platform powered by a lakehouse brings together the best of healthcare data lake and data warehouse architectures. With this strong foundation, healthcare organizations can analyze and act on data to build tailored solutions and serve stakeholders efficiently.