How to safeguard your healthcare data journey with data seatbelts
Managing healthcare data can feel like a risky endeavor. Just as drivers aim for safety, unforeseen circumstances can lead to accidents. In Arcadia’s byte-sized insight series, Mary Kuchenbrod, VP of Data Operations, explains how to approach data management as a safety-first journey. She discusses how healthcare data platforms utilize data strategies commonly used in cybersecurity and the military to protect your organization. This empowers you to stay intact from the impact of data mishaps, ensuring stability and continuity in the face of unexpected challenges.
Data management strategies to prepare for unforeseen events
Within a healthcare data platform, safety precautions can be put in place to minimize damage in the event of a data crash, or what can be referred to as the “boom.” When something goes wrong, you want users to continue using the platform uninterrupted.
Kuchenbrod divvies this up into two phases: left of boom and right of boom — a concept originating from the military and later adopted by cybersecurity. Left of boom strategies focus on preemptive measures while right of boom kick in post-incident.
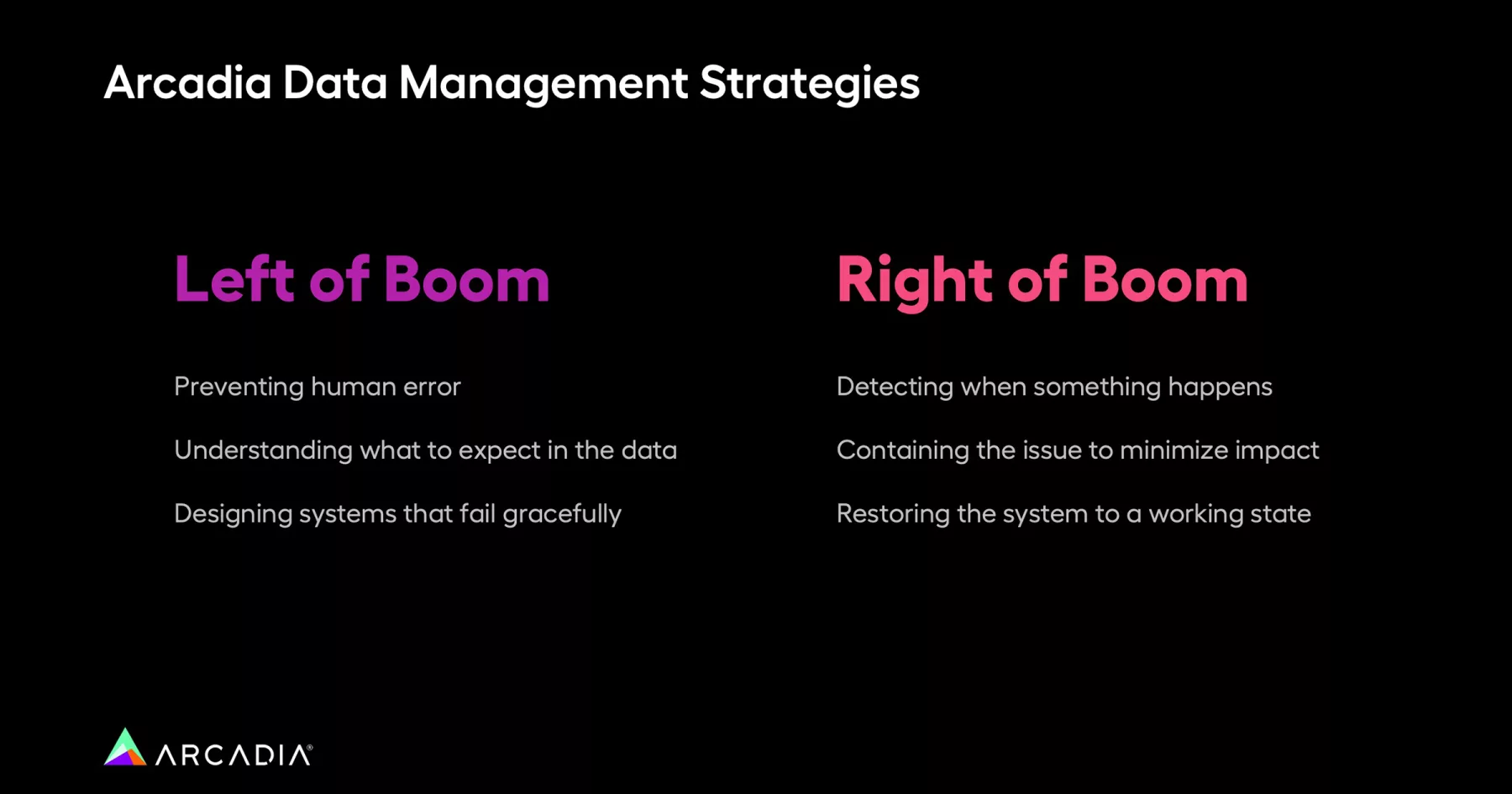
Left of boom asks: what preventive actions can you take to keep your systems safe? In data management, the key left of boom considerations include:
- Preventing human error: Are we automating repeatable tasks, and do we have clear operating procedures and checklists to reduce mistakes?
- Understanding of what to expect in the data: How do we make sure users have a solid foundation of what right looks like?
- Designing systems that fail gracefully: What is the reaction of your system and its ability to see data as it comes in, and stops correct data from being held up?
After the “boom” has occurred, right of boom activities kick in. Because healthcare data is messy, with a fragmented ecosystem, right of boom can be a common occurrence. Here are the most important considerations after the “boom,” something like missing data in this case, happens:
- Detecting when something happens: What are your abilities to constantly monitor inbound data?
- Containing the issue to minimize impact: Once something has gone wrong, how do you catch and isolate it?
- Restoring the system to a working state: When you notice something has gone wrong, how quickly can recover back to standard operations?
The most important right of boom strategy is containing the issue to minimize its impact and keep data operations moving smoothly until its fixed. This is something Mary refers to as a “data seatbelt.”
Data seatbelts from 2-point to 4-point
Seatbelts are a useful analogy because we have different types depending on the risk level of an activity. On an airplane, you might just use a 2-point lap belt, but an infant in a car needs something more secure like a 6-point safety harness.
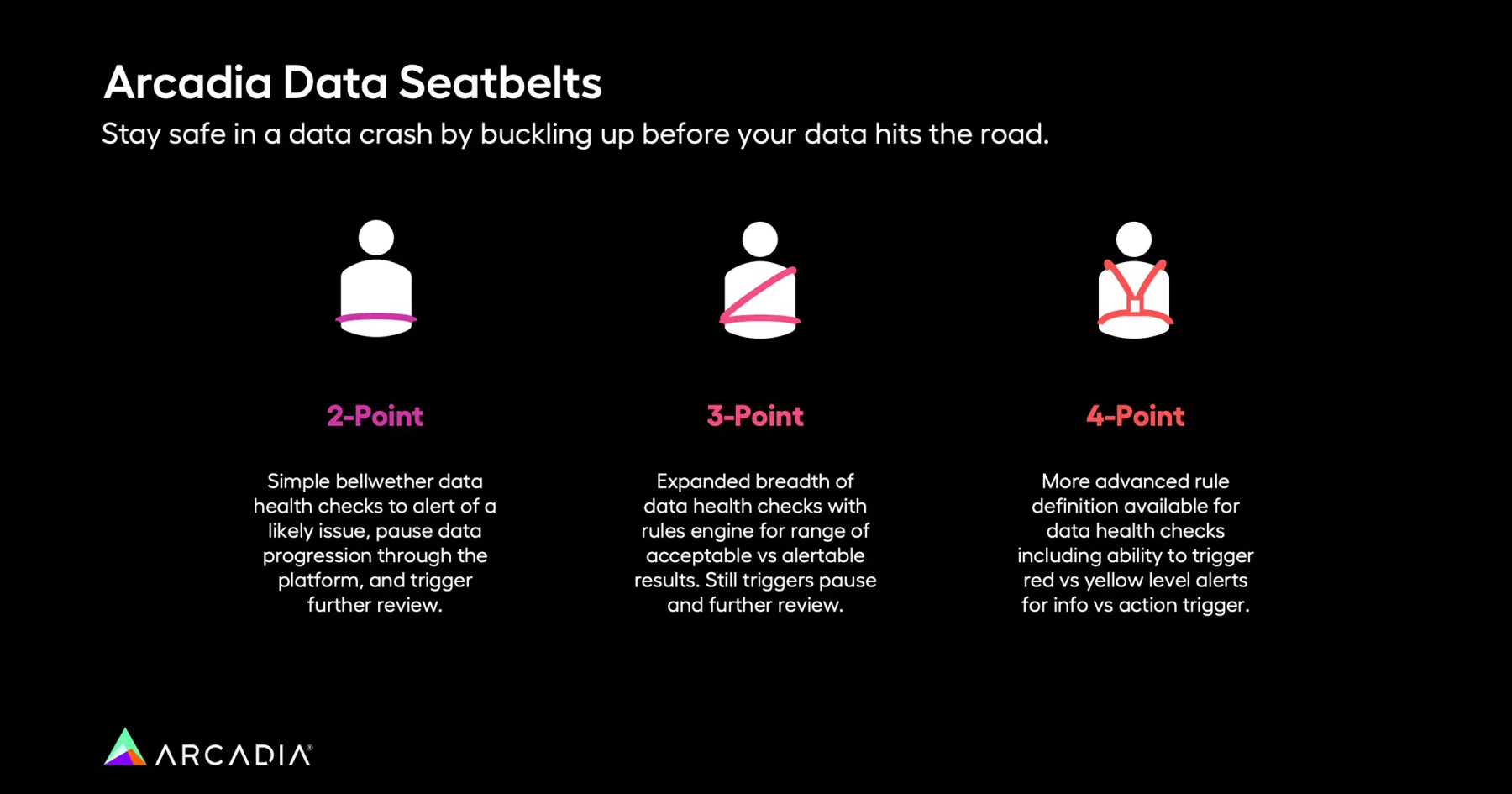
There is a spectrum of how heavy-handed you’d like to be with your data seatbelts. We’re going to focus on 2-point, 3-point, and 4-point seatbelts:
- The 2-Point seatbelt introduces basic yet essential health checks, pausing for review at the hint of an issue
- The 3-Point seatbelt expands the breadth of these checks, incorporating a rules engine to discern between acceptable and alert-worthy results with a more nuanced response
- The 4-Point seatbelt offers advanced rule definitions for data checks, distinguishing between critical alerts and informational flags, fine-tuning the response to data incidents
Each tier allows organizations to choose the level of protection that best suits their needs.
Ensuring data quality and integrity with real-world applications
Data seatbelts are not a one-size-fits-all solution but a comprehensive suite of measures tailored to various aspects of data integrity. Now that we’ve covered the spectrum of data seatbelts, let’s define three use cases: quality measures, risk gap profiles, and claims data monitoring. We’ll explore what causes “booms” for each and consider the best data seatbelts based on varying levels of risk tolerance.
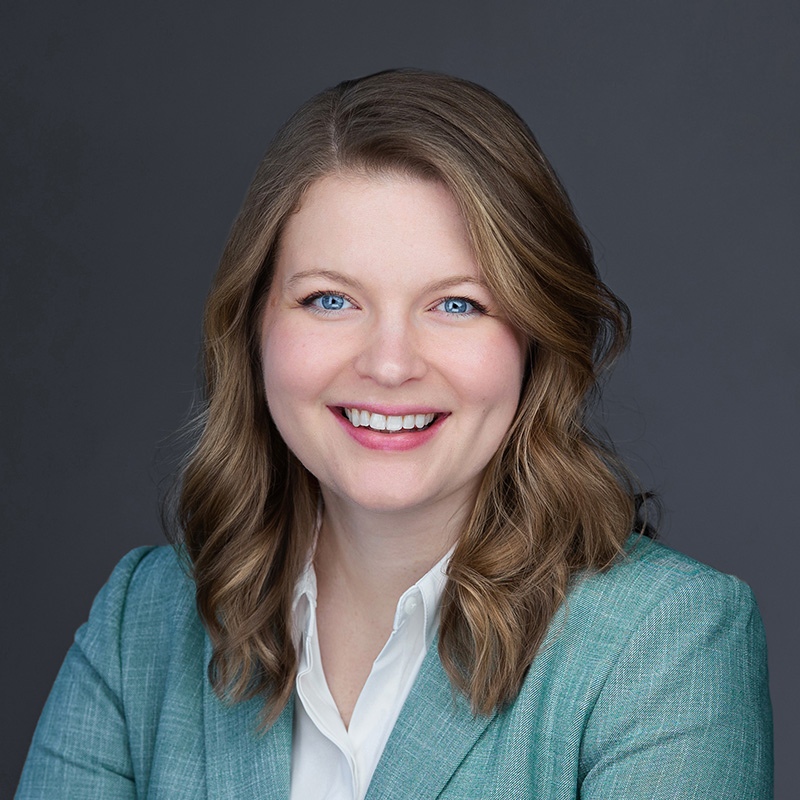
Quality measures is the first use case I go to because they have an extremely complex set of relationships and dependencies with the upstream data that goes into calculating a quality measure.
Quality measures
Upstream data issues can introduce major inaccuracies in measure results. Once you export measures from the measure engine, they propagate downstream, breaking workflows and sometimes taking days to revert. For a 2-point quality measure seatbelt, select one or a small number of bellwether measures. Automatically run pre- and post-comparison of denominators and numerators across adjacent measure runs, set thresholds which will kill the job before export, and set email addresses to receive alerts.
For a 3-point seatbelt in this case, expand from bellwether measures to all measures in the system. Measures whose prior comparison baseline had fewer than 1000 denominator hits are excluded. This case is expected to have more “noise” relative to “signal” and require more capacity for frequent reviews.

Risk gap profiles
Like quality measures, the number of gaps associated with patient profiles in any given program are sensitive to upstream data issues. When issues occur, how do you prevent end users from getting impacted?
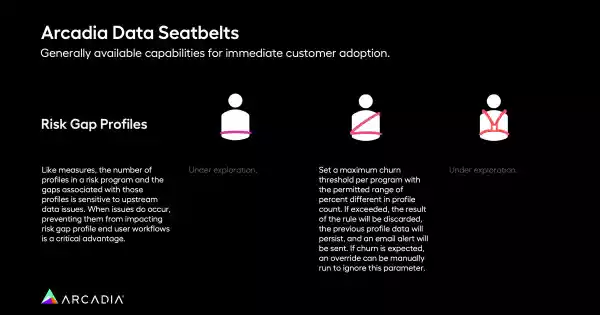
In this case a 2-point data seatbelt would set a limit for how much the number of profiles can change in each program. If the change is too big, the system will ignore the new data and keep using old data. If you know that the number of profiles is going to change by a lot, you can turn off this limit manually. This maintains trust and stability even in the face of data anomalies. This type of check is commonly run by default in software like Arcadia’s Assess.
Claims data monitoring
Claims datasets are essential for analytics in value-based care. Ensuring the quality of data is vital for maintaining its integrity. By thoroughly comparing and evaluating claims data, we can prevent inaccuracies and maintain high standards. This preemptive quality control is crucial in the monitoring process, confirming data reliability prior to integration with a data platform.

In this scenario, a 4-point seatbelt can be utilized with a full comparison of member months, PMPMs, and select data quality rule outputs on every claims data package, every month. Data does not progress to load to your data platform until it has been reviewed by a human and approved for use.
Securing your data for the road ahead
Data seatbelts offer a robust framework to ensure data integrity and security. Adopting these innovative measures is key to safeguarding your healthcare data.
"Data crashes happen. Make sure you're wearing a seatbelt," says Kuchenbrod. These seatbelts ensure users are held safe in the event something goes wrong.
Watch more byte-sized insights or request a demo to learn how we can safeguard your data, together.