Exploratory data analysis for long-COVID’s healthcare impact
What drives long-COVID? This analysis helps us understand the complex interactions using real-world data.
As many as 1 in 10 people may have symptoms of COVID-19 for months after their initial infection, driving a staggering total number of long-COVID cases that continues to increase. As part of our work with the COVID Patient Recovery Alliance, Arcadia analyzed a massive real-world data set to identify potential drivers of long-COVID.
Our key finding is that vaccination against COVID-19 reduces the likelihood that patients will experience long-COVID symptoms in the event that they are infected. Our data also suggest that a single dose of a COVID-19 vaccine, even if received after the patient is diagnosed with COVID, still correlates with a reduced likelihood of presenting long-COVID symptoms. We’ve intended this summary of our analysis to be useful for a broad healthcare audience; for more detailed clinical or technical information about our COVID-19 work and research data asset, see our report on MedRxiv.
I bring to your attention the fact that a number of individuals who virologically have recovered from [their COVID-19] infection, in fact have persistence measured in weeks to months of symptomatology that does not appear to be due to persistence of the virus. They’re referred to as long haulers. They have fatigue, myalgia, fever, and involvement of the neurological system, as well as cognitive abnormalities, such as the inability to concentrate.
— Dr. Anthony “Tony” Fauci, Testimony to the Senate HELP Committee, September 23, 2020
Our study Reduced Incidence of Long-COVID Symptoms Related to Administration of COVID-19 Vaccines Both Before COVID-19 Diagnosis and Up to 12 Weeks After (Michael A. Simon, Ryan D. Luginbuhl, Richard Parker) is currently available on MedRxiv.
What is long-COVID?
The COVID Patient Recovery Alliance defines long-COVID as the currently-accepted term to describe signs and symptoms that continue or develop after acute COVID-19 that are not explained by an alternative diagnosis.
It includes both ongoing symptomatic COVID-19 as well as post-COVID-19 syndrome, the onset and persistence of COVID symptoms during and after infection.
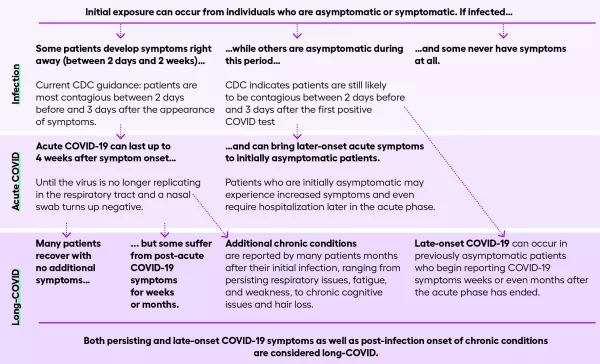
Long-COVID is a critical research priority for the COVID Patient Recovery Alliance, which brings together key thought leaders in business, health care, research, academia, data and analytics, and patient advocacy to develop national solutions that coordinate diverse data sources, inform the development of models of care, and ensure adequate payment for long-COVID patients who served their communities and nation during the pandemic; whose COVID-19 related costs are extraordinary and burdensome; or who are underserved by existing programs.
Arcadia is a contributor to the Alliance, lending our research data asset and data science expertise to this important cause.
What happens once a patient is diagnosed with COVID-19?
The journey varies. Some remain asymptomatic. Others develop immediate symptoms (gastrointestinal, cardiovascular, and/or others) and then quickly recover — or may be hospitalized. Some only begin to develop symptoms weeks after diagnosis. And for many, COVID symptoms persist for many weeks and may seem unending. There is no single long-COVID experience. Patients suffer from a wide range of symptoms over highly variable timeframes. The complex nature of this condition makes it challenging to identify potential drivers — but data science can offer some insights. See the data visualization.
Exploring factors driving long-COVID
Arcadia used exploratory data analysis to surface factors that might increase patient susceptibility to long-COVID symptoms. Exploratory data analysis does not assign causal relationships, but it does help clarify complex interactions and drive hypothesis creation. Our findings identify strong directions for productive future research.
Our analysis involved the following practical, concrete steps, which can be applied to other problems where you need to understand potential relationships between complex interactions using a massive real-world data set.
1. Analyze patient conditions
We established baselines for patients who had been diagnosed and treated for COVID-19 using a broad spectrum of chronic and acute conditions, both COVID associated and not. Understanding the prevalence of chronic conditions in the populations we studied helped us characterize the incidence of COVID-related symptoms and conditions as patients recovered.
2. Select a data set
We needed a real-world data set that not only had a sufficient population of COVID-19 patients but also represented a more general population of active patients to support an exploratory analysis. Arcadia’s research data asset includes de-identified, HIPAA-compliant RWD on a growing active patient population. At the time this study was conducted, our data included 1.3 million COVID-19 positive patients of whom ~689,000 had data from an electronic medical records (EMR) system.
Arcadia’s COVID-19 Population as of January 2022
- 2.8M COVID-19 positive patients
- 2.4M with data from an EMR (88%)
- 8.9M COVID-19 vaccinated patients
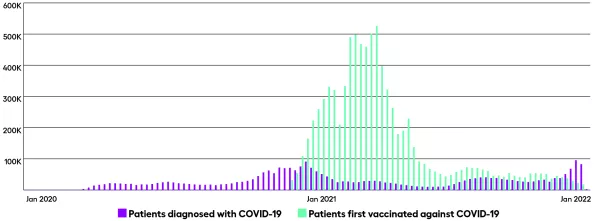
3. Define and refine cohorts
We included 25.8 million patients of any age who were seen clinically at least once prior to January 1, 2020 and were alive as of March 1, 2020. Of those, our COVID positive (COV+) group included 1.06 million patients with a positive COVID-19 PCR or antigen test or a diagnosis by a provider in a clinical setting. Both our COV+ patients and the 24.7 million patient control group (COV-) had diverse racial and ethnic representation.
4. Classify conditions
A “condition” is a clinical diagnosis that associates a disease, sign, or symptom with a patient at a point in time. We used the AHRQ Chronic Condition Indicators to split out chronic and acute events. We also used AHRQ’s clinical classification system to define broad diagnostic groupings. We then identified COVID-associated conditions (using value sets of COVID-associated conditions courtesy of Clinical Architecture with their permission) that superseded these broad diagnostic groupings.
5. Insert events of interest
In addition to diagnostic criteria, we added other events sourced from EHR or claims-based data that we thought might be useful to our model, like hospitalizations related to a COVID diagnosis, vitals and labs, medications, and COVID-19 vaccinations.
6. Understand timing and condition onset
Looking at events the week they occurred (rather than the date) allowed us to simplify our view and compress the data without reducing their value.
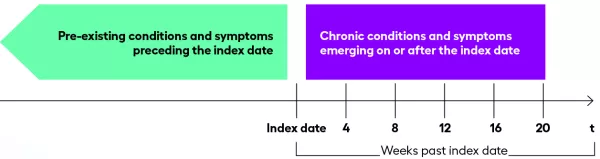
7. Analyze prevalence
We looked at the prevalence of conditions present prior to our study period (and verified the data against existing literature) to create baselines for the COV+ group and the COV- control group. Here, we started to see some qualitative differences between the groups — for example, COV+ patients were 11.7% more likely to suffer from obesity.
8. Analyze incidence
As we continued to work through our analysis, we needed to figure out what separated long-COVID patients from the COV+ group and from the COV-control group.
Incidence counts indicate the onset of new or acute conditions or symptoms. By analyzing shifts in incidence counts relative to the timing of COVID diagnosis and treatment, we established a baseline. We then compared the baselines for the COV+ group against clusters that have experienced long-COVID symptoms.
Why exploratory data analysis?
Exploratory data analysis is particularly well-suited to pulling out hidden insights about complex interactions. By analyzing the symptomatic journeys of more than 1 million COVID patients, our model helped us surface factors that strongly correlate with whether a patient develops long-COVID.
We used our research data asset that was built to support the needs of healthcare organizations taking on value-based care. Insights generated from the full spectrum of healthcare data support research into both common conditions like diabetes and rare diseases like spinal muscular atrophy (SMA).
Our long-COVID findings
The COVID data and our initial analysis support the hypothesis that vaccination reduces the odds of experiencing long-COVID symptoms, even if the vaccination occurs after COVID-19 infection.
- Patients who are vaccinated and then infected are 4–6 times less likely to experience long-COVID symptoms and 7–10 times less likely to report more than one symptom.
- Patients who are first vaccinated after they are diagnosed with COVID-19 are still less likely to experience multiple long-COVID symptoms compared to patients who remain unvaccinated: 4-6 times less likely if vaccinated within a month after diagnosis and 3 times less likely if vaccinated within two months of diagnosis.
- The protective effect of a vaccine administered after infection declines within 2 months of infection.
Vaccines reduce the likelihood of developing long-COVID
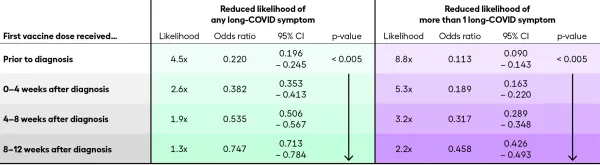
What are the key findings from this study?
These findings were an unexpected and exciting development from Arcadia’s ongoing work with the COVID-19 Patient Recovery Alliance. The most important takeaways are:
- COVID vaccines not only reduce the incidence and terrible effects of acute COVID infection but they also reduce the likelihood and severity of long-COVID symptoms in the aftermath of that infection.
- These findings offer evidence that vaccination soon after infection is still highly protective against long-COVID, extending the window of opportunity for the unvaccinated to protect themselves against long-COVID symptoms.
- The protective effect of vaccination against long-COVID increases the personal protection offered by vaccination to the recipient, which may encourage increased prophylactic vaccination and, with continued support from evidence, therapeutic vaccination.
What else can we learn about long-COVID from our massive real-world dataset?
A massive RWD dataset like Arcadia’s permits us to explore a number of additional avenues of study, including:
- The differences in long-COVID experiences based on demographic factors, including age, sex, race, ethnicity, and socioeconomic status.
- The impact of pre-existing conditions on presence and severity of long-COVID symptoms, including the onset of new chronic conditions.
- Differences in long-COVID symptoms for patients treated with different medications and therapeutics during the acute phase of their COVID infection.
- Variation in expression of long-COVID symptoms based on combinations of likely COVID variant and vaccination status.
- Impact of multiple COVID infections and vaccinations on long-COVID, especially as their effects on the immune system continue to rise in importance.
We are grateful for the opportunity to better serve our customers and contribute work to address a monumental public health crisis, and we look forward to continuing our work with the COVID-19 Patient Recovery Alliance.