Impact and challenges of large language models in healthcare
Large language models could revolutionize the way organizations deliver healthcare and drive patient engagement. When trained on robust health datasets, these models can play a crucial role in enhancing patient care through data-driven insights.
In this guide, learn about the potential and challenges of large language models in healthcare, and how organizations can experiment responsibly with this powerful tool.
The basics of large language models in healthcare
Large language models (LLMs) are deep learning models trained on a large neural network. In essence, LLMs can take a large sequence of text and figure out what it means — fast. So, it makes sense that LLMs are transforming various industries, processing vast amounts of information quickly and freeing up employees’ time for other important tasks.
When applied to healthcare, large language models could offer innovative solutions for improving patient care and streamlining processes. Let’s examine the potential for LLMs in healthcare.
What is the role of large language models in medicine?
LLMs can answer questions, summarize text, paraphrase complicated jargon, and translate words into different languages. From quickly scanning a long patient file to helping patients understand the real-world ramifications of their diagnosis, a tool that can slice through huge chunks of language could revolutionize the way we give and receive care.
What are the use cases of medical LLMs?
Medical providers can rely on large language models in healthcare to:
- Streamline administrative tasks: Clinicians spend roughly 33% of their workday on activities outside of patient care, such as administrative requirements. LLMs can streamline workflows to reduce time spent scheduling appointments, sending follow-up care instructions, or completing other administrative tasks.
- Manage clinical documentation: LLMs can summarize patient notes and medical histories, allowing providers to quickly draw meaningful insights from patient data and develop effective treatment plans.
- Proactively detect adverse events: Using data from electronic health records (EHRs), LLMs may be able to automatically detect adverse health events.
Still, like any new technology, integrating this capability in a healthcare environment presents unique challenges.
The challenges of implementing LLMs in healthcare
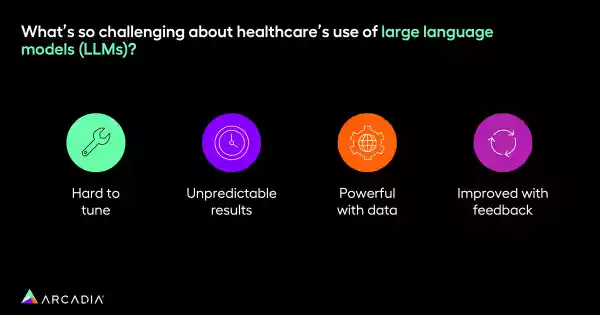
Before investing in AI or LLMs, healthcare organizations should be aware of the potential challenges. Most commonly, these are:
Complexity in fine-tuning
Fine-tuning large language models for healthcare purposes is a complex task. LLMs are essentially vast neural networks, containing an immense graph of interconnected facts and weights. While many general-purpose LLMs have some healthcare-related knowledge, they also pull from external information, including biases and consumer behaviors.
Brendan Smith-Elion, VP of Product Management at Arcadia, describes these models as “a giant sea of facts.” This vast pool of data can lead to unintended interference, where irrelevant or misleading information finds its way into the output.
The only way to avoid this is through meticulous tuning and adjustment, which lets healthcare professionals filter out data that’s wrong or unhelpful and ensure the models produce accurate and relevant healthcare insights.
Unpredictable results due to information drift
LLMs constantly receive new information, which can lead to a phenomenon known as information or model drift.
Unlike traditional healthcare AI and machine learning systems where data control is more manageable, LLMs in healthcare — especially those provided by large commercial entities — are subject to constant changes in their knowledge base. This drift can make it difficult to maintain accuracy and reliability, as the underlying information the model relies on can shift over time.
Dependence on extensive contextual data
LLMs function effectively when they’re supplied with extensive contextual data. The accuracy of an LLM’s output relates to the amount and relevance of the data from which it draws. However, injecting such a high level of detailed data into LLMs is often challenging out-of-the-box.
We must develop effective strategies and tactics to provide adequate context for these models for specific healthcare use cases. Without this, the models may fail to deliver the desired precision and relevance in their responses.
Necessity of a feedback loop
Establishing a feedback loop is crucial for refining outcomes and continuously enhancing model performance. By incorporating user feedback — such as ratings or actions — into the model’s training cycle, healthcare organizations can enhance the accuracy and usefulness of the outputs over time. This iterative process of refinement, sometimes called a “virtuous loop,” is necessary to adapt the LLM so it meets user needs and is reliable in healthcare settings.
4 practical steps for fine-tuning medical LLMs
Healthcare has been slower to adopt advanced AI technologies compared to other sectors due to concerns over data privacy, the high cost of infrastructure, and the critical nature of healthcare decisions. Working with users to develop trust and transparency will be an important part of overcoming this hesitation.
The key to building trust with LLMs in healthcare is a continuous improvement process where users provide regular feedback that helps refine the model. The “Plan, Do, Study, Act” system offers a structured framework for implementing and refining AI systems in healthcare:
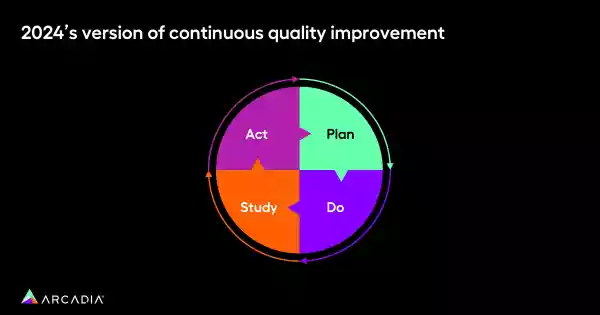
1. Plan: Create an implementation plan
By planning ahead, healthcare organizations can maximize the benefits they receive from an LLM and streamline the implementation process:
- Build a task inventory for your users
- Build an automation opportunity grid and rank each job based on data readiness, user trust in AI, relative infrastructure cost, and adoption of existing behavioral levers
“For each of those opportunities, think about how ready your data is: Is the data available? Is it clean? Is it trusted? Is it actionable?” Smith-Elion says. Finally, multiply each value and rank your opportunities in order of feasibility.
2. Do: Experiment with LLMs
With your key opportunities identified, “experiment,” Smith-Elion says. “Figure out what makes sense.” For instance, healthcare organizations can:
- Leverage free LLMs, like Gemini or ChatGPT, to test use cases and gather example output which can pick up on potential issues or pitfalls early on
- Build experimental frameworks that generate qualitative and quantitative feedback
- Use the RLHF (Reinforcement Learning from Human Feedback) method to improve the tools with user feedback, suppressing unhelpful results that would erode user trust
- Combine human-curated responses with high-cost model outputs to train lower-cost, fine-tuned models
The combination of the RLHF method and AI will sharpen the LLM’s accuracy and usefulness.
3. Study: Assess your model’s results
Once you tune and adjust your model, you can assess and rank its results. Incorporating clinicians in this phase will help you refine the model and gather more useful feedback from end users. Start by taking the following steps:
- Hold an expert review of tuned output via a stack rank based on criteria relevant to your use case
- Train the model based on the stack rank output, pushing in expert clinical thinking
- Repeat until variance in expert-ranked output is negligible for your use case
The more expert clinical thinking an organization pushes in, the less variance in quality it will find in an LLM’s output. “It’s important to have clinician and nursing input, folks that are relevant to the use case, providing that feedback to you,” Smith-Elion says.
4. Act: Collect user feedback
Finally, set up mechanisms to collect user feedback from all end users:
- Ensure there is a way to get user feedback, both passive and active
- Audit the randomized output with a clinical expert panel. Evaluate it for efficacy, model drift, and potential bias
- Build in automated, model-tuning processes based on RLHF
- Flag dead-end responses where the user did not complete a job (i.e., patient did not schedule a referral)
Keep in mind that something as simple as a thumbs up or down button will help organizations refine their LLM, maintaining user trust. A clinical expert panel can audit randomized output, so there’s always a finger on the pulse.
Automate, innovate, and collaborate with LLMs
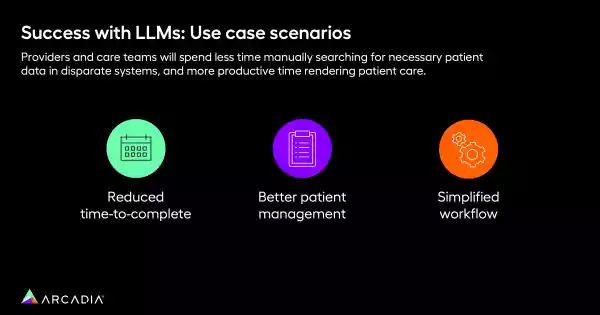
With a thoughtful plan in place for their use, LLMs could revolutionize healthcare. Already, they can reduce the time required to complete manual tasks, and their ability to summarize complicated information could make a huge impact on patient management.
Simplifying workflows through automation could save healthcare organizations a vast amount of time and resources. As technology advances, organizations can expect even more sophisticated AI tools that offer greater precision and efficiency.
Get in on the ground floor of LLMs in healthcare. As technology develops, healthcare can embrace it with the appropriate safeguards in place, leveraging it now while planning for AI’s potential. Learn how you can foster a tech-forward environment that improves workflows, outcomes, and savings.